{R} Dictionary-based Approach to Populist Style: Evidence from the DIPMS Database
<< The content below was replaced by this entry. |
1 Introduction
This is Markdown document of the article in preparation “Aping the People”: A Text Analysis of Populist Mimesis in Narendra Modi Speeches. Results presented are preliminary: they are subject to substantial change as the analyis is currently being refined ahead of submission.
How are the dictionaries validated?
- Though using internal consistency reliability (Chronbach Alpha test with psych R package) https://www.rdocumentation.org/packages/psych/versions/1.8.12
- Through contextualising the dictionaries using two similar procedures:
-Word embedding: https://quanteda.io/articles/pkgdown/replication/text2vec.html
-Target word-collocation: https://tutorials.quanteda.io/advanced-operations/target-word-collocations/
2 Preparing the corpus for analysis
knitr::opts_chunk$set(warning=FALSE, message=FALSE)
#remove(list = ls())
#Change column numbers
dictnumber=37
#load packages
library(quanteda)
library(readtext)
library(ggplot2)
library(lubridate)
library(devtools)
library(robustHD)
library(dplyr)
library(DescTools)
library(multcomp)
library(multcompView)
library(sjstats)
library(stm)
library(lda)
library(topicmodels)
library(igraph)
library(textometry)
library(ca)
library(FactoMineR)
library(car)
library(tseries)
library(stringi)
library(bbmle)
library(ngram)
library(text2vec)
Preparation: Step 1
A dataframe (i.e. spreadsheet) called “dataframe” is created. CSV and text files are put together in the folder test_dataX. Texts and CSVs are UTF8 ‘TXM’ formatted, CSV comma separated].
setwd("C:/Users/jtmartelli/Google Drive/Textual_analysis/R/aping")
txtvars <-read.csv("metadata.csv",stringsAsFactors = FALSE)
bodytexts <-readtext('*.txt')
#In case of need, unecessary features of the speech can be removed (not run)
#bodytexts2 <- gsub("http:.*$", "", bodytexts2) # replace just the urls/http/www part
#bodytexts2 <- gsub("https:.*$", "", bodytexts2) # replace just the urls/http/www part
#bodytexts2 <- gsub("www.*$", "", bodytexts2) #
#bodytexts2 <- gsub("http:.*", "", bodytexts2) # replace all of the urls
#bodytexts2 <- gsub("https:.*", "", bodytexts2) # replace all of the urls
#bodytexts2 <- gsub("www.*", "", bodytexts2) #
#bodytexts2 <- gsub("[[:punct:]]", "", bodytexts2) # remove all punctuation
#bodytexts2 <- gsub("[^\x20-\x7F\x0D\x0A]", "", bodytexts2) # remove all non-ascii characters, https://stackoverflow.com/questions/38182860/what-is-the-best-way-to-remove-non-ascii-characters-from-a-text-corpus-when-usin
#bodytexts2 <- gsub("[0-9]", "", bodytexts2) # remove numbers
#bodytexts2 <- gsub("^\\s+|\\s+$", "", bodytexts2) # remove extra leading and trailing whitespace
#bodytexts2 <- tolower(bodytexts2) # turn all letters lower case
bodytexts$id<-gsub('.txt','',bodytexts$doc_id)
dataframe<-merge(bodytexts,txtvars,by='id')
Preparation: Step 2
A corpus (i.e. documents & metadata) called “workcorpus” is generated from the dataframe “dataframe.”
For more on of the command, c.f. https://tutorials.quanteda.io/basic-operations/corpus/corpus/.
workcorpus <- corpus(dataframe)
texts(workcorpus) <- iconv(texts(workcorpus), from = "UTF-8", to = "ASCII", sub = "")
#summary(workcorpus)
#Debugging in further corpuses [1]: workcorpus <- corpus(dataframe, enc = NULL, encTo = "UTF-8")
#Debugging in further corpuses [2]: dataframe2 <- na.omit(dataframe)
#For convenience: if needed it is possible to rename document IDs. Below is an example.
#docid <- paste(dataframe$loc,
#dataframe$year,
#dataframe$no, sep = " ")
#docnames(workcorpus) <- docid
#summary(workcorpus, 5)
Preparation: Step 3
A sub-corpus called “subworkcorpus” is created from the corpus “workcorpus” in order to analyze the relevant section of the corpus. For more on of the command, c.f. https://tutorials.quanteda.io/basic-operations/corpus/corpus_subset/.
#head(docvars(workcorpus))
subworkcorpus<-corpus_subset(workcorpus, format %in% c('speech')) #to restrict to speeches #if to restrict to pmo speeches, then add the following: & period %in% c('pmo')
ndoc(subworkcorpus)
## [1] 4156 |
Preparation: Step 4
The sub-corpus “subworkcorpus” is tokenized. NB: A function concatenate words can be plugged for N-Grams.
For more on of the command, c.f. https://tutorials.quanteda.io/basic-operations/tokens/tokens/.
tokssubworkcorpus <- tokens(subworkcorpus, remove_punct = FALSE, remove_numbers = FALSE, remove_symbols = FALSE, remove_separators = TRUE, remove_hyphens = FALSE, remove_url = FALSE, concatenator = "_")
head(tokssubworkcorpus[[1]], 50)
Preparation: Step 5
The couple of unigrams proxying entries of variables of interest are replaced by ngrams. For more on of the command, c.f. https://github.com/quanteda/quanteda/issues/1022 and https://rdrr.io/cran/quanteda/man/tokens_lookup.html.
gsub go over loops to replace item one of culum 2 into item 1 of column one and so on and so forth.
popngrams <- read.csv("C:/Users/jtmartelli/Google Drive/Textual_analysis/R/aping/dictionaries/popngrams.csv", as.is = TRUE, header = FALSE)
#popngrams[,1]
#popngrams[,2]
dicopopgram <- dictionary(split(popngrams[,2], popngrams[,1]))
ngramstokssubworkcorpus <- tokens_lookup(tokssubworkcorpus, dicopopgram, valuetype = 'glob', exclusive = FALSE, capkeys = FALSE, case_insensitive = FALSE)
Preparation: Step 6
A document-feature matrix DFM (i.e. a TXM index with row and columns pivoted) is created from the tokenized corpus.
For more on the command, c.f. https://tutorials.quanteda.io/basic-operations/dfm/dfm/.
dfmtokssubworkcorpus <- dfm(ngramstokssubworkcorpus, remove_punct = FALSE, tolower = FALSE, dictionary_regex=TRUE, language = "english", stem = FALSE, clean = FALSE, verbose= TRUE) # if no ngram dictionary is loaded, then used the insert tokssubworkcorpus instead.
Preparation: Step 7
Group the DFM per Prime Ministers (the default in the csv is speech-wise).
dfmperpm <- dfm_group(dfmtokssubworkcorpus, groups = "loc") #ndoc(dfmperpm) #nfeat(dfmperpm) topfeatures(dfmperpm)
![]() |
#docfreq(dfmperpm[,c("on","the")])
#topfeatures(dfmperpm[,c('on','the')])
#textstat_frequency(dfmperpm[,c('on','the')])
#textstat_frequency(dfmperpm)
dfmperyear <- dfm_group(dfmtokssubworkcorpus, groups = "year")
dfmperday <- dfm_group(dfmtokssubworkcorpus, groups = "day")
3 The analysis
Dictionaries
A dictionary of populist style vocabulary containing three-level hierarchical entries. For more on the command, c.f. https://kenbenoit.net/assets/courses/tcd2018qta/assignment3_LASTNAME_FIRSTNAME.html, https://www.oipapio.com/question-1382670.
#Step 1:
#Import the dictionaries. There are two types of dicos: a hierarchical matrix and a flat list one.
popdicoH <- dictionary(file = "C:/Users/jtmartelli/Google Drive/Textual_analysis/R/aping/dictionaries/populism.yml", tolower = FALSE)
names(popdicoH) #that is the matrix one
popdicoL <- dictionary(list(populism=c("elit*","consensus*","undemocratic","referend*","corrupt","propaganda","politici*","*deceit*","*deceiv*","*betray*","shame","scandal*","truth*","dishonest*","establishm*","ruling*"))) #that is the list-wise dictionary using Pauwels list.
#Step2:
#Applying the dictionary to the DFM
#Unweighted dictionary
#popdicodfmHpm is the unweighted dictionary matrix if corpus is organised PM-wise (3 levels)
popdicodfmHpm <- dfm_lookup(dfmperpm, dictionary = popdicoH, levels=3)
head(popdicodfmHpm)
![]() |
#popdicodfmHspeech is the unweighted dictionary matrix if corpus is organised speech-wise (3 levels)
popdicodfmHspeech <- dfm_lookup(dfmtokssubworkcorpus, dictionary = popdicoH, levels=3)
head(popdicodfmHspeech)
#popdicodfmHspeech is the unweighted dictionary matrix if corpus is organised speech-wise (2 levels)
popdicodfmHspeech <- dfm_lookup(dfmtokssubworkcorpus, dictionary = popdicoH, levels=2)
topfeatures(popdicodfmHspeech)
print(popdicodfmHspeech)
#popdicodfmHspeech is the unweighted dictionary list (x1) if corpus is organised speech-wise (1 levels)
popdicodfmHspeech <- dfm_lookup(dfmtokssubworkcorpus, dictionary = popdicoH, levels=1)
topfeatures(popdicodfmHspeech)
print(popdicodfmHspeech)
#Weighted dictionary (PM wise)
#popdicodfmHpmWeight is the weighted dictionary matrix if corpus is organised PM-wise (3 levels)
dfmperpmw <- dfm_weight(dfmperpm,"prop")
popdicodfmHpmWeight <- dfm_lookup(dfmperpmw, dictionary = popdicoH, levels=3)
#popdicodfmHpmWeight is the weighted dictionary matrix if corpus is organised PM-wise (2 levels)
popdicodfmHpmWeighttwolevels <- dfm_lookup(dfmperpmw, dictionary = popdicoH, levels=2)
#popdicodfmHpmWeight is the weighted dictionary matrix if corpus is organised PM-wise (2 levels)
popdicodfmHpmWeightonelevel <- dfm_lookup(dfmperpmw, dictionary = popdicoH, levels=1)
#Weighted dictionary (Speech-wise)
#popdicodfmHspeechWeight is the weighted dictionary matrix if corpus is organised speech-wise (three levels)
weightdfmtokssubworkcorpus<-dfm_weight(dfmtokssubworkcorpus,"prop")
popdicodfmHspeechWeight <- dfm_lookup(weightdfmtokssubworkcorpus, dictionary = popdicoH, valuetype = "glob", levels=3)
#popdicodfmHspeechWeighttwolevels is the weighted dict matrix of speech-wise corpus (two levels)
popdicodfmHspeechWeighttwolevels <- dfm_lookup(weightdfmtokssubworkcorpus, dictionary = popdicoH, valuetype = "glob", levels=2)
#popdicodfmHspeechWeightonelevel is the weighted dict matrix of speech-wise corpus (one level)
popdicodfmHspeechWeightonelevel <- dfm_lookup(weightdfmtokssubworkcorpus, dictionary = popdicoH, valuetype = "glob", levels=1)
#popdicodfmLspeechWeightonelevel is the weighted dict list of speech-wise corpus (one level) from Pauwels (2011)
popdicodfmLspeechWeightonelevel <- dfm_lookup(weightdfmtokssubworkcorpus, dictionary = popdicoL, valuetype = "glob")
#popdicoHflat <-flatten_dictionary(popdicoH)
#popdicoHflat$populist.intimacy.family
#compare <- dfm(weightdfmtokssubworkcorpus, dictionary = popdicoH, valuetype = "glob")
#compare2 <- dfm_lookup(weightdfmtokssubworkcorpus, dictionary = popdicoH, valuetype = "glob", levels=3)
Standard Deviation
Here are computed the mean standard deviations of the PMs for each linguistic measure.
##PART 1: with all the sub sub variables
popdicodfmHpmDF <- convert(popdicodfmHpm, to = "data.frame")
#If the standard deviation is kept unweighted:
#Step1: conversion of the unweighted dictionary into a data frame
#popdicodfmHpmDF <- convert(popdicodfmHpm, to = "data.frame")
#Step2: After pulling out the first sub-sub-variable, compute the standard deviation for each PM.
#populist.intimacy.family
#familyU<-popdicodfmHpmDF$populist.intimacy.family #the old command before levelling-up the dictionary
familyU<-popdicodfmHpmDF$family
familymean<-mean(familyU)
familySD<-sd(familyU)
standardisechandrafamilyU<-(familyU[1]-familymean)/familySD
#standardisechandrafamilyU
#standardisechanranfamilyU<-(familyU[2]-familymean)/familySD
#standardisechanranfamilyU
#standardisedesaifamilyU<-(familyU[3]-familymean)/familySD
#standardisedesaifamilyU
#standardiseindirafamilyU<-(familyU[4]-familymean)/familySD
#standardiseindirafamilyU
#standardisemmsfamilyU<-(familyU[5]-familymean)/familySD
#standardisemmsfamilyU
#standardisemodifamilyU<-(familyU[6]-familymean)/familySD
#standardisemodifamilyU
#standardisenehrufamilyU<-(familyU[7]-familymean)/familySD
#standardisenehrufamilyU
#standardiserajivfamilyU<-(familyU[8]-familymean)/familySD
#standardiserajivfamilyU
#standardiseraofamilyU<-(familyU[9]-familymean)/familySD
#standardiseraofamilyU
#standardisevajpayeefamilyU<-(familyU[10]-familymean)/familySD
#standardisevajpayeefamilyU
#standardisevpsinghfamilyU<-(familyU[11]-familymean)/familySD
#standardisevpsinghfamilyU
#populist.intimacy.emotion
#emotionU<-popdicodfmHpmDF$emotion
#familymean<-mean(familyU)
#familySD<-sd(familyU)
#for(i in 1:37)#all columns
#{
#FreqValues<-as.numeric(as.character(popdicodfmHpmWeight[,i]))
#!!!!! DO THAT WITH THE OTHER SUB-SUB-VARIABLES OF THE DICTIONARY WHEN FINALISED !!!!!
#If the standard deviation is weighted:
#Step1: conversion of the weighted dictionary into a data frame
popdicodfmHpmWeightDF <- convert(popdicodfmHpmWeight, to = "data.frame")
#Step2: After pulling out the first sub-sub-variable, compute the standard deviation for each PM.
#populist.intimacy.family #dictionrary was levelled down to $family
#familyW<-popdicodfmHpmWeightDF$family
#familymean<-mean(familyW)
#familySD<-sd(familyW)
#standardisechandrafamilyW<-(familyW[1]-familymean)/familySD
#standardisechandrafamilyW
#standardisechanranfamilyW<-(familyW[2]-familymean)/familySD
#standardisechanranfamilyW
#standardisedesaifamilyW<-(familyW[3]-familymean)/familySD
#standardisedesaifamilyW
#standardiseindirafamilyW<-(familyW[4]-familymean)/familySD
#standardiseindirafamilyW
#standardisemmsfamilyW<-(familyW[5]-familymean)/familySD
#standardisemmsfamilyW
#standardisemodifamilyW<-(familyW[6]-familymean)/familySD
#standardisemodifamilyW
#standardisenehrufamilyW<-(familyW[7]-familymean)/familySD
#standardisenehrufamilyW
#standardiserajivfamilyW<-(familyW[8]-familymean)/familySD
#standardiserajivfamilyW
#standardiseraofamilyW<-(familyW[9]-familymean)/familySD
#standardiseraofamilyW
#standardisevajpayeefamilyW<-(familyW[10]-familymean)/familySD
#standardisevajpayeefamilyW
#standardisevpsinghfamilyW<-(familyW[11]-familymean)/familySD
#standardisevpsinghfamilyW
##Looping the weighted standard deviations across whole data set
ColumnNames<-names(popdicodfmHpmWeightDF)
StanValue<-array(NA,dim=c(11,dictnumber+1)) #If I change the number of columns change 38 to x
StanValue[,1]<-popdicodfmHpmWeightDF[,1] #if nb of column changes, don't touch
for(wordtype in 2:(dictnumber+1))#Column for loop,
{
WordMean<-mean(popdicodfmHpmWeightDF[,wordtype])
WordStanDev<-sd(popdicodfmHpmWeightDF[,wordtype])
for(primemin in 1:11)#Row for loop
{
WeigthedPMValue<-popdicodfmHpmWeightDF[primemin,wordtype]
StandardisedValue<-round((WeigthedPMValue-WordMean)/WordStanDev,digits=2)
StanValue[primemin,wordtype]<-StandardisedValue
}#end prime minister loop
}#end word type loop
colnames(StanValue)<-ColumnNames
#write.csv(StanValue,file="")
#head(StanValue)
StanValue[1:11,]
library(data.table)
StanValueDF<-as.data.frame(StanValue)
#populist.intimacy.emotion
#emotionW<-popdicodfmHpmWeightDF$emotionaltone
#emotionW
#!!!!! DO THAT WITH THE OTHER SUB-SUB-VARIABLES OF THE DICTIONARY WHEN FINALISED !!!!!
##PART 2: with all the sub variables
popdicodfmHpmWeighttwolevelsDF <- convert(popdicodfmHpmWeighttwolevels, to = "data.frame")
ColumnNames2<-names(popdicodfmHpmWeighttwolevelsDF)
popdicodfmHpmWeighttwolevelsDF
StanValue2<-array(NA,dim=c(11,4)) #If I change the number of columns change 38 to x
StanValue2[,1]<-popdicodfmHpmWeighttwolevelsDF[,1] #if nb of column changes, don't touch
for(wordtype2 in 2:4)#Column for loop,
{
WordMean2<-mean(popdicodfmHpmWeighttwolevelsDF[,wordtype2])
WordStanDev2<-sd(popdicodfmHpmWeighttwolevelsDF[,wordtype2])
for(primemin2 in 1:11)#Row for loop
{
WeigthedPMValue2<-popdicodfmHpmWeighttwolevelsDF[primemin2,wordtype2]
StandardisedValue2<-round((WeigthedPMValue2-WordMean2)/WordStanDev2,digits=2)
StanValue2[primemin2,wordtype2]<-StandardisedValue2
}#end prime minister loop
}#end word type loop
colnames(StanValue2)<-ColumnNames2
#write.csv(StanValue,file="")
#head(StanValue)
StanValue2[1:11,]
library(data.table)
StanValue2DF<-as.data.frame(StanValue2)
##PART 3: with the variable (populism, 1 level, dictionary under study)
popdicodfmHpmWeightonelevelDF <- convert(popdicodfmHpmWeightonelevel, to = "data.frame")
ColumnNames3<-names(popdicodfmHpmWeightonelevelDF)
PopulismW<-popdicodfmHpmWeightonelevelDF$Populism
PopulismWmean<-mean(PopulismW)
PopulismSD<-sd(PopulismW)
PopulismW
PopulismSD
sdPopulismW<-c()
for (o in 1:11){
sdPopulismW <- c(sdPopulismW,(PopulismW[o]-PopulismWmean)/PopulismSD)
}
sdPopulismW<-round(sdPopulismW,digits=2)
StanValue3<-sdPopulismW
#colnames(StanValue3)<-ColumnNames3
##PART 4: compare the results with Pauwels' dictionary
popdicodfmLspeechWeightonelevelDF <- convert(popdicodfmLspeechWeightonelevel, to = "data.frame")
ColumnNames4<-names(popdicodfmLspeechWeightonelevelDF)
PopulismWL<-popdicodfmLspeechWeightonelevelDF$populism
PopulismWLmean<-mean(PopulismWL)
#PopulismWLmean
PopulismLSD<-sd(PopulismWL)
#PopulismLSD
sdPopulismWL<-c()
for (p in 1:11){
sdPopulismWL <- c(sdPopulismWL,(PopulismWL[p]-PopulismWLmean)/PopulismLSD)
}
sdPopulismWL<-round(sdPopulismWL,digits=2)
StanValue4<-sdPopulismWL
#colnames(StanValue4)<-ColumnNames4
Plot: First outputs
Here the relative frequencies of the dictionary entries are plotted.
#tabletitle<-c("A","B","C","D","E","F","G","H","I")
tabletitle<-ColumnNames[-1] #titles
tabletitle[1]<-"Institutional Processes"#Just changes first
#tabletitle<-c("Institutional Processes","Political Parties")#Change all by putting names in the vector as text. NB: it will work only if we have a vector of all 37 entries.
colourchart<-c("darkorange","cyan3","brown1","burlywood","blue","red","chartreuse4","chocolate3","green","black","darkorchid")
#plotm<-matrix(c(1:37),nrow=7,ncol=6,byrow=TRUE)
#layout(plotm,heights=c(0.3,0.3,0.3,0.3,0.3,0.3,0.3))
RowOrder<-c(7,4,3,2,8,11,1,9,10,5,6)
#for(i in c(3,5,7,9)) #If I want to pull out specific columns
for(i in 1:dictnumber)#all columns
{
FreqValues<-as.numeric(as.character(popdicodfmHpmWeight[,i]))
par(mar=c(0.5,2,1,1))
plot(1:length(FreqValues),FreqValues,mgp = c(3, 1, 0),xaxt='n',xlab="",ylab="Relative Frequency",main=tabletitle[i],type='n',las=0,ylim=c(0,max(FreqValues)))
#axis(2,at=c(0,max(FreqValues)),labels=c(0,round(max(FreqValues),digits=2)))
for(k in 1:11)
{
segments(k,0,k,FreqValues[RowOrder[k]],lwd=4,col=colourchart[k])
}
}

plot(1,1,type="n",axes=FALSE,xlab="",ylab="")##empty plot for legend
legend(x="top",inset=0,
legend=c("J.Nehru","I.Gandhi","M.Desai","C.Singh","R.Gandhi","VP.Singh","C.Shekhar","PVN.Rao","AB.Vajpayee","M.Singh","N.Modi"),
col=colourchart,lwd=5,cex=1,horiz=FALSE) #size of the legend
Anova 1, Post-Hoc & Effect Sizes
Here the one-way anova is conducted on the relevant linguistic measures to examine differences between Prime Ministers. For more on the command, c.f. https://www.sthda.com/english/wiki/one-way-anova-test-in-r. [1] For more on size effect, c.f. https://cran.r-project.org/web/packages/sjstats/vignettes/anova-statistics.html.
#PART 1: With the sub-sub variables of the dictionary of the article (weighted, 3 levels)
#Step 1: Anova
pm <- as.factor(popdicodfmHspeechWeight@docvars$loc)
#head(pm)
resultanova = aov(as.matrix(popdicodfmHspeechWeight)~as.factor(pm)) #check caps & regex once
summary.aov(resultanova)

#str(summary(resultanova)) summary.aov(resultanova)[[13]][["F value"]][[1]] #F value extracted from column 13
#Step 2: Pulling out F values fvaluelists<-c() for (f in 1:dictnumber){ fvaluelists <- c(fvaluelists,summary.aov(resultanova)[[f]][["F value"]][[1]]) } fvaluelists<-round(fvaluelists,digits=2) #fvaluelists <- c(summary.aov(resultanova)[[1]][["F value"]][[1]],summary.aov(resultanova)[[2]][["F value"]][[1]],summary.aov(resultanova)[[3]][["F value"]][[1]],summary.aov(resultanova)[[4]][["F value"]][[1]],summary.aov(resultanova)[[5]][["F value"]][[1]],summary.aov(resultanova)[[6]][["F value"]][[1]],summary.aov(resultanova)[[7]][["F value"]][[1]],summary.aov(resultanova)[[8]][["F value"]][[1]],summary.aov(resultanova)[[9]][["F value"]][[1]],summary.aov(resultanova)[[10]][["F value"]][[1]],summary.aov(resultanova)[[11]][["F value"]][[1]],summary.aov(resultanova)[[12]][["F value"]][[1]],summary.aov(resultanova)[[13]][["F value"]][[1]]...till 37) #Step 3: Running the effect sizes estimates over every column of the dictionrary popdicodfmHspeechWeightasdf <- convert(popdicodfmHspeechWeight, to = "data.frame") resultanovapm<-c() for(i in 2:(dictnumber+1)) { anovatemp<-aov(popdicodfmHspeechWeightasdf[,i]~as.factor(pm)) correctiontemp<-eta_sq(anovatemp) correctionnumeric<-round(as.numeric(as.character(correctiontemp[2])),digits=2) resultanovapm<-c(resultanovapm,correctionnumeric) } resultanovapm
#Step 4: Putting the Anova and effect sizes at the end of the standard deviation table fvaluesfordf<-c("F*",fvaluelists) anovaresultsfordf<-c("n^2",resultanovapm) Table1<-rbind(StanValue,fvaluesfordf,anovaresultsfordf) Table1
colnames(Table1)[1]<-"Prime Minister" #Changes the first column name
#colnames(pretable1)<-c("Prime Minister","Institutional Processes") #chages all the column names by putting all 37 in a vector. NB: it will work only if we have a vector of all 37 entries.
Table1DF<-as.data.frame(Table1,row.names=FALSE) #convert to data frame
Table1DF
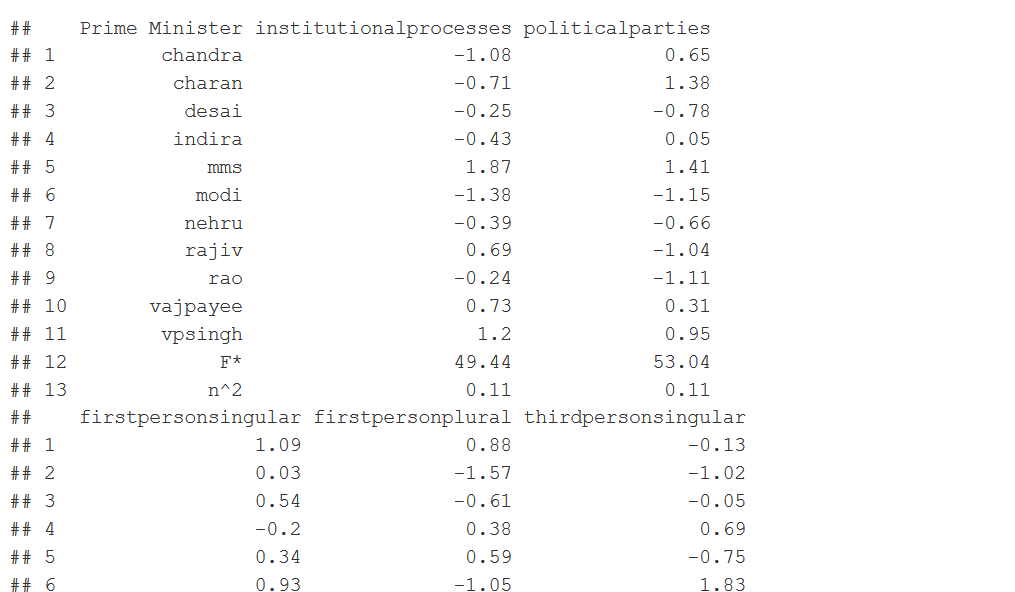
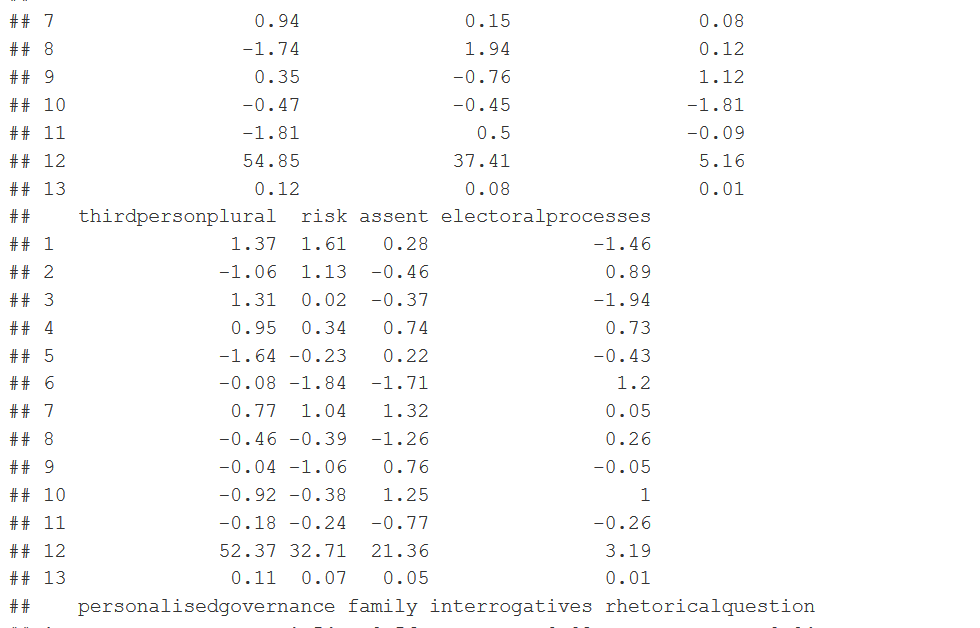
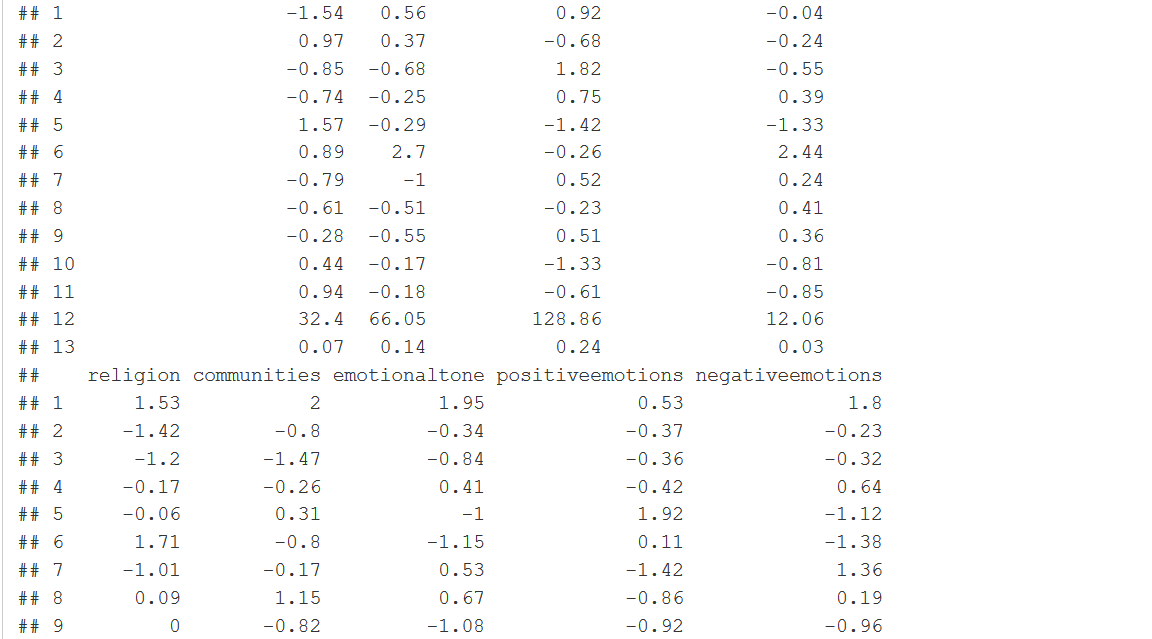
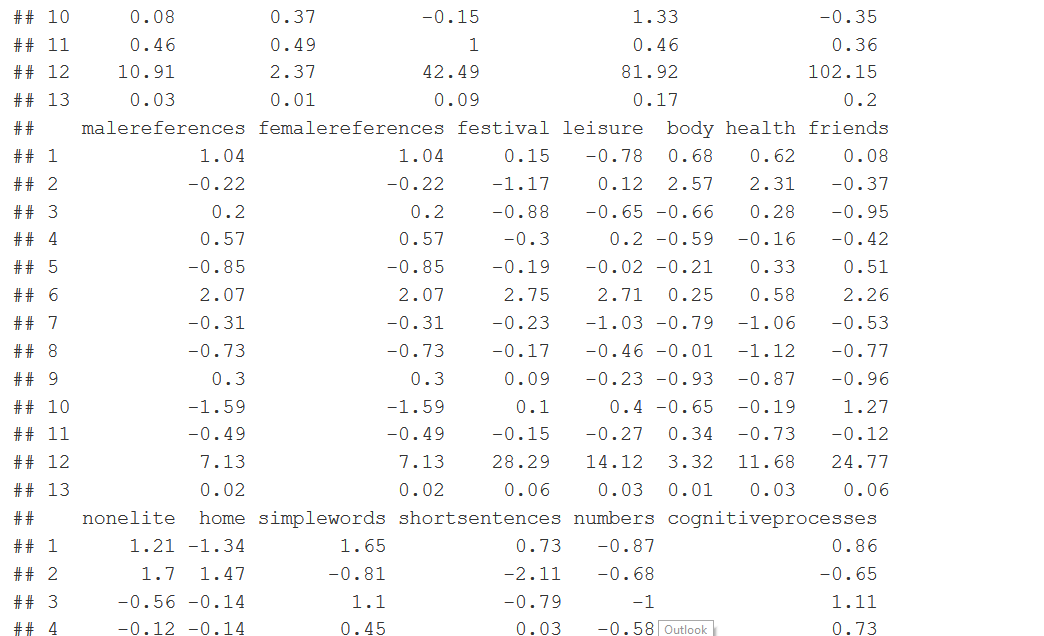
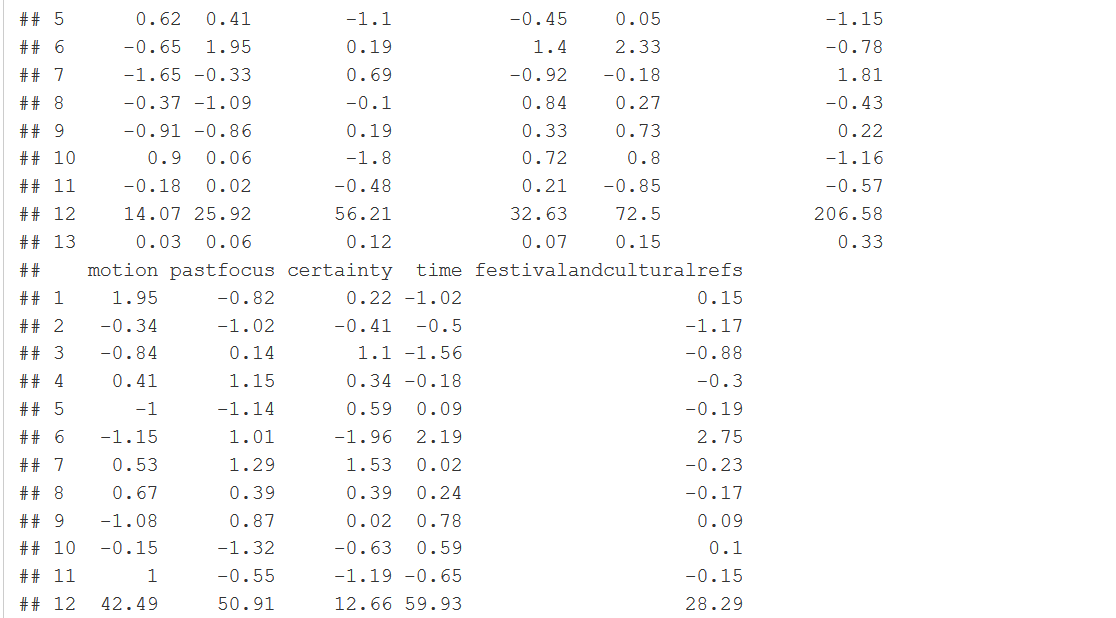
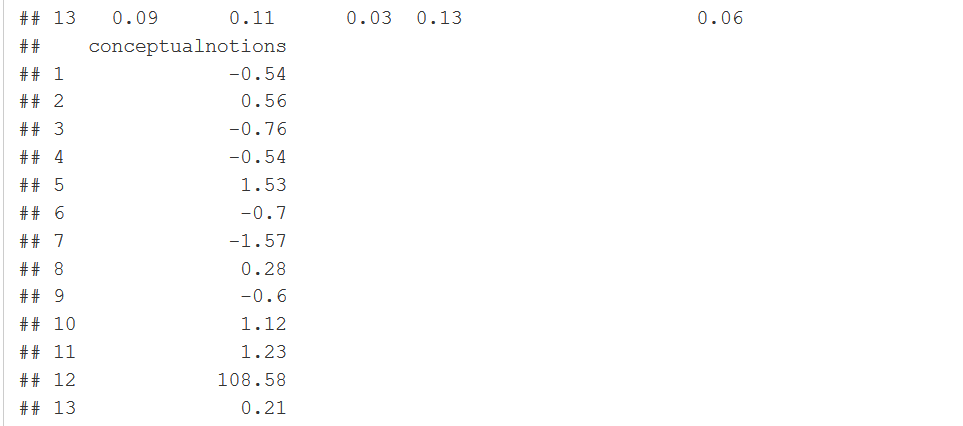
#PART 2: With the sub variables of the dictionary of the article (weighted, 2 levels)
pm2 <- as.factor(popdicodfmHspeechWeighttwolevels@docvars$loc)
head(pm2)
resultanova2 = aov(as.matrix(popdicodfmHspeechWeighttwolevels)~as.factor(pm2)) #check caps & regex once
summary.aov(resultanova2)
summary.aov(resultanova2)[[2]][["F value"]][[1]] #F value extracted from column 2
#Step 2: Pulling out F values
fvaluelists2<-c()
for (g in 1:3){
fvaluelists2 <- c(fvaluelists2,summary.aov(resultanova2)[[g]][["F value"]][[1]])
}
fvaluelists2<-round(fvaluelists2,digits=2)
#Step 3: Running the effect sizes estimates over every column of the dictionrary
popdicodfmHspeechWeighttwolevelsdf <- convert(popdicodfmHspeechWeighttwolevels, to = "data.frame")
resultanovapm2<-c()
for(h in 2:4)
{
anovatemp2<-aov(popdicodfmHspeechWeighttwolevelsdf[,h]~as.factor(pm2))
correctiontemp2<-eta_sq(anovatemp2)
correctionnumeric2<-round(as.numeric(as.character(correctiontemp2[2])),digits=2)
resultanovapm2<-c(resultanovapm2,correctionnumeric2)
}
resultanovapm2
#Step 4: Putting the Anova and effect sizes at the end of the standard deviation table
fvaluesfordf2<-c("F",fvaluelists2)
anovaresultsfordf2<-c("n^2",resultanovapm2)
Table2<-rbind(StanValue2,fvaluesfordf2,anovaresultsfordf2)
colnames(Table2)[1]<-"Prime Minister" #Changes the first column name
#colnames(pretable1)<-c("Prime Minister","Institutional Processes") #chages all the column names by putting all 37 in a vector. NB: it will work only if we have a vector of all 37 entries.
Table2DF<-as.data.frame(Table2,row.names=FALSE) #convert to data frame
Table2DF
#PART 3: With the populist dictionary, compared to the Pauwels' one
#sdPopulismW
#sdPopulismWL
#Step 1: The populist dictionary under study
pm3 <- as.factor(popdicodfmHspeechWeightonelevel@docvars$loc)
head(pm3)
resultanova3 = aov(as.matrix(popdicodfmHspeechWeightonelevel)~as.factor(pm3)) #check caps & regex once
summary.aov(resultanova3)
fvaluelist3<-summary.aov(resultanova3)[[1]][["F value"]][[1]]
fvaluelist3
resultanovapm3<-eta_sq(resultanova3)
fvaluelist3<-round(fvaluelist3,digits=2)
result<-round(resultanovapm3$etasq,digits=2)
fvaluesfordf3<-c("F",fvaluelist3)
anovaresultsfordf3<-c("n^2",result)
NewStan<-array(c(Table1[c(-12,-13),1],StanValue3),dim=c(11,2))
Table3<-rbind(NewStan,fvaluesfordf3,anovaresultsfordf3)
colnames(Table3)<-c("Prime Minister","Tested Dictionary")#Changes the first column name
#colnames(pretable1)<-c("Prime Minister","Institutional Processes") #chages all the column names by putting all 37 in a vector. NB: it will work only if we have a vector of all 37 entries.
Table3DF<-as.data.frame(Table3,row.names=FALSE) #convert to data frame
Table3DF
#Step 2: Pauwel's dictionary
pm4 <- as.factor(popdicodfmLspeechWeightonelevel@docvars$loc)
head(pm4)
resultanova4 = aov(as.matrix(popdicodfmLspeechWeightonelevel)~as.factor(pm4)) #check caps & regex once
summary.aov(resultanova4)
resultanova4$coefficients
fvaluelist4<-summary.aov(resultanova4)[[1]][["F value"]][[1]]
fvaluelist4
resultanovapm4<-eta_sq(resultanova4)
fvaluelist4<-round(fvaluelist4,digits=2)
result2<-round(resultanovapm4$etasq,digits=2)
fvaluesfordf4<-c("F",fvaluelist4)
anovaresultsfordf4<-c("n^2",result2)
NewStan2<-array(c(Table1[c(-12,-13),1],StanValue4),dim=c(11,2))
Table4 <- rbind(NewStan2,fvaluesfordf4,anovaresultsfordf4)
colnames(Table4)<-c("Prime Minister","Pauwel Dictionary")#Changes the first column name
#colnames(pretable1)<-c("Prime Minister","Institutional Processes") #chages all the column names by putting all 37 in a vector. NB: it will work only if we have a vector of all 37 entries.
Table4DF<-as.data.frame(Table4,row.names=FALSE) #convert to data frame
Table4DF
#Step 3: Column-bind of the two dictionaries
Table5 <- cbind(Table3,Table4[,2])
colnames(Table5)<-c("Prime Minister","Tested Dictionary","Pauwel's Dictionary")
Table5DF<-as.data.frame(Table5,row.names=FALSE)
Table5DF
Inverse Relashionships
Here dictionary sub sub variables that express inverse relashionships with the phenomenon at hands are multiplied by -1.
#Part 1: Straighten inverse relashionships of the pm-wise dictionary
#Step 1: Turn inverse relashionships into straight relashionships
ChangeVector<-c(-1,-1,1,-1,1,1,-1,-1,1,1,1,1,1,1,1,1,1,1,1,1,1,1,1,1,1,1,1,1,1,1,-1,-1,1,-1,1,1,-1)
NewStanValue<-array(NA,dim=c(11,(dictnumber+1)))
NewStanValue[,1]<-as.character(StanValueDF[,1])
for(i in 2:(dictnumber+1))
{
ColumnPull<-as.numeric(as.character(StanValueDF[,i]))
NewColumn<-ColumnPull*ChangeVector[i-1]
NewStanValue[,i]<-NewColumn
}
colnames(NewStanValue)<-ColumnNames
NewStanValueDF<-as.data.frame(NewStanValue)
#Step 2: Set up matrix of sub variables by grouping together sub-sub variables of interests
NewMatrix<-array(NA,dim=c(11,4))
NewMatrix[,1]<-NewStanValue[,1]#prime ministers names are the first column
NewMatrix[,2]<-round(as.numeric(NewStanValue[,2])+as.numeric(NewStanValue[,3])+as.numeric(NewStanValue[,4])+as.numeric(NewStanValue[,5])+as.numeric(NewStanValue[,6])+as.numeric(NewStanValue[,7])+as.numeric(NewStanValue[,10])+as.numeric(NewStanValue[,11])+as.numeric(NewStanValue[,34]),digits=2) #Deintermediation
NewMatrix[,3]<-round(as.numeric(NewStanValue[,8])+as.numeric(NewStanValue[,9])+as.numeric(NewStanValue[,12])+as.numeric(NewStanValue[,13])+as.numeric(NewStanValue[,15])+as.numeric(NewStanValue[,17])+as.numeric(NewStanValue[,18])+as.numeric(NewStanValue[,19])+as.numeric(NewStanValue[,20])+as.numeric(NewStanValue[,21])+as.numeric(NewStanValue[,23])+as.numeric(NewStanValue[,24])+as.numeric(NewStanValue[,25])+as.numeric(NewStanValue[,26])+as.numeric(NewStanValue[,28])+as.numeric(NewStanValue[,33])+as.numeric(NewStanValue[,36]),digits=2) #Intimacy
NewMatrix[,4]<-round(as.numeric(NewStanValue[,14])+as.numeric(NewStanValue[,16])+as.numeric(NewStanValue[,22])+as.numeric(NewStanValue[,27])+as.numeric(NewStanValue[,29])+as.numeric(NewStanValue[,30])+as.numeric(NewStanValue[,31])+as.numeric(NewStanValue[,32])+as.numeric(NewStanValue[,35])+as.numeric(NewStanValue[,37])+as.numeric(NewStanValue[,38]),digits=2) #Cognitive Simplicity
head(NewMatrix)
#Step 3: Set up matrix of the variable 'populism' but grouping all the sub-sub variables
NewMatrix2<-array(NA,dim=c(11,2))
NewMatrix2[,1]<-NewStanValue[,1]#prime ministers names are the first column
for(j in 1:11)#row loop
{
rowsum=0
for(i in 2:(dictnumber+1))#column loop
{
rowsum<-rowsum+as.numeric(NewStanValue[j,i])
}#end column loop
NewMatrix2[j,2]<-round(rowsum,digits=2)
}#end row loop
NewMatrix2 #Task 2, replicate the procedure for text-wise/date-wise dictionary
#Part 2: Straighten inverse relashionships of the speech-wise dictionary
#Step 1: a speech-wise matrix with the mean standard deviations of the means is generated
popdicodfmHspeechWeightDF <- convert(popdicodfmHspeechWeight, to = "data.frame")
ColumnNames5<-names(popdicodfmHspeechWeightDF)
StanValue5<-array(NA,dim=c(4156,dictnumber+1))
StanValue5[,1]<-popdicodfmHspeechWeightDF[,1]
for(wordtypeS in 2:(dictnumber+1))#Column for loop,
{
WordMeanS<-mean(popdicodfmHspeechWeightDF[,wordtypeS])
WordStanDevS<-sd(popdicodfmHspeechWeightDF[,wordtypeS])
for(primeminS in 1:4156)#Row for loop
{
WeigthedSpeechValueS<-popdicodfmHspeechWeightDF[primeminS,wordtypeS]
StandardisedValueS<-round((WeigthedSpeechValueS-WordMeanS)/WordStanDevS,digits=2)
StanValue5[primeminS,wordtypeS]<-StandardisedValueS
}#end speech loop
}#end word type loop
StanValue5DF<-as.data.frame(StanValue5)
#Step 2: Turn inverse relashionships into straight relashionships
ChangeVector<-c(-1,-1,1,-1,1,1,-1,-1,1,1,1,1,1,1,1,1,1,1,1,1,1,1,1,1,1,1,1,1,1,1,-1,-1,1,-1,1,1,-1)
NewStanValue5<-array(NA,dim=c(4156,dictnumber+1))
NewStanValue5[,1]<-as.character(StanValue5DF[,1])
for(i in 2:(dictnumber+1))
{
ColumnPull<-as.numeric(as.character(StanValue5DF[,i]))
NewColumn<-ColumnPull*ChangeVector[i-1]
NewStanValue5[,i]<-NewColumn
}
colnames(NewStanValue5)<-ColumnNames
NewStanValue5DF<-as.data.frame(NewStanValue5)
#Step 3: Set up matrix of sub variables by grouping together sub-sub variables of interests
NewMatrix3<-array(NA,dim=c(4156,4))
NewMatrix3[,1]<-NewStanValue5[,1]#text names names are the first column
NewMatrix3[,2]<-round(as.numeric(NewStanValue5[,2])+as.numeric(NewStanValue5[,3])+as.numeric(NewStanValue5[,4])+as.numeric(NewStanValue5[,5])+as.numeric(NewStanValue5[,6])+as.numeric(NewStanValue5[,7])+as.numeric(NewStanValue5[,10])+as.numeric(NewStanValue5[,11])+as.numeric(NewStanValue5[,34]),digits=2) #Deintermediation
NewMatrix3[,3]<-round(as.numeric(NewStanValue5[,8])+as.numeric(NewStanValue5[,9])+as.numeric(NewStanValue5[,12])+as.numeric(NewStanValue5[,13])+as.numeric(NewStanValue5[,15])+as.numeric(NewStanValue5[,17])+as.numeric(NewStanValue5[,18])+as.numeric(NewStanValue5[,19])+as.numeric(NewStanValue5[,20])+as.numeric(NewStanValue5[,21])+as.numeric(NewStanValue5[,23])+as.numeric(NewStanValue5[,24])+as.numeric(NewStanValue5[,25])+as.numeric(NewStanValue5[,26])+as.numeric(NewStanValue5[,28])+as.numeric(NewStanValue5[,33])+as.numeric(NewStanValue5[,36]),digits=2) #Intimacy
NewMatrix3[,4]<-round(as.numeric(NewStanValue5[,14])+as.numeric(NewStanValue5[,16])+as.numeric(NewStanValue5[,22])+as.numeric(NewStanValue5[,27])+as.numeric(NewStanValue5[,29])+as.numeric(NewStanValue5[,30])+as.numeric(NewStanValue5[,31])+as.numeric(NewStanValue5[,32])+as.numeric(NewStanValue5[,35])+as.numeric(NewStanValue5[,37])+as.numeric(NewStanValue5[,38]),digits=2) #Cognitive Simplicity
head(NewMatrix3)
#Step 4: Set up matrix of the variable 'populism' but grouping all the sub-sub variables
NewMatrix4<-array(NA,dim=c(4156,2))
NewMatrix4[,1]<-StanValue5[,1]#prime ministers names are the first column
for(j in 1:4156)#row loop
{
rowsum=0
for(i in 2:(dictnumber+1))#column loop
{
rowsum<-rowsum+as.numeric(NewStanValue5[j,i])
}#end column loop
NewMatrix4[j,2]<-round(rowsum,digits=2)
}#end row loop
#NewMatrix4
#Part 3: Straighten inverse relashionships of the year-wise dictionary
#Step 1: a speech-wise matrix with the mean standard deviations of the means is generated
popdicodfmHpmYWeight <- dfm_lookup(dfmperyear, dictionary = popdicoH, valuetype = "glob", levels=3)
popdicodfmHpmYWeightDF <- convert(popdicodfmHpmYWeight, to = "data.frame")
ColumnNames6<-names(popdicodfmHpmYWeightDF)
StanValue6<-array(NA,dim=c(71,dictnumber+1))
StanValue6[,1]<-popdicodfmHpmYWeightDF[,1]
for(wordtypeY in 2:(dictnumber+1))#Column for loop,
{
WordMeanY<-mean(popdicodfmHpmYWeightDF[,wordtypeY])
WordStanDevY<-sd(popdicodfmHpmYWeightDF[,wordtypeY])
for(primeminY in 1:71)#Row for loop
{
WeigthedSpeechValueY<-popdicodfmHpmYWeightDF[primeminY,wordtypeY]
StandardisedValueY<-round((WeigthedSpeechValueY-WordMeanY)/WordStanDevY,digits=2)
StanValue6[primeminY,wordtypeY]<-StandardisedValueY
}#end speech loop
}#end word type loop
StanValue6DF<-as.data.frame(StanValue6)
#Step 2: Turn inverse relashionships into straight relashionships
ChangeVector<-c(-1,-1,1,-1,1,1,-1,-1,1,1,1,1,1,1,1,1,1,1,1,1,1,1,1,1,1,1,1,1,1,1,-1,-1,1,-1,1,1,-1)
NewStanValue6<-array(NA,dim=c(71,dictnumber+1))
NewStanValue6[,1]<-as.character(StanValue6DF[,1])
for(i in 2:(dictnumber+1))
{
ColumnPull<-as.numeric(as.character(StanValue6DF[,i]))
NewColumn<-ColumnPull*ChangeVector[i-1]
NewStanValue6[,i]<-NewColumn
}
colnames(NewStanValue6)<-ColumnNames
NewStanValue6DF<-as.data.frame(NewStanValue6)
#Step 3: Set up matrix of sub variables by grouping together sub-sub variables of interests
NewMatrix5<-array(NA,dim=c(71,4))
NewMatrix5[,1]<-NewStanValue6[,1]#text names names are the first column
NewMatrix5[,2]<-round(as.numeric(NewStanValue6[,2])+as.numeric(NewStanValue6[,3])+as.numeric(NewStanValue6[,4])+as.numeric(NewStanValue6[,5])+as.numeric(NewStanValue6[,6])+as.numeric(NewStanValue6[,7])+as.numeric(NewStanValue6[,10])+as.numeric(NewStanValue6[,11])+as.numeric(NewStanValue6[,34]),digits=2) #Deintermediation
NewMatrix5[,3]<-round(as.numeric(NewStanValue6[,8])+as.numeric(NewStanValue6[,9])+as.numeric(NewStanValue6[,12])+as.numeric(NewStanValue6[,13])+as.numeric(NewStanValue6[,15])+as.numeric(NewStanValue6[,17])+as.numeric(NewStanValue6[,18])+as.numeric(NewStanValue6[,19])+as.numeric(NewStanValue6[,20])+as.numeric(NewStanValue6[,21])+as.numeric(NewStanValue6[,23])+as.numeric(NewStanValue6[,24])+as.numeric(NewStanValue6[,25])+as.numeric(NewStanValue6[,26])+as.numeric(NewStanValue6[,28])+as.numeric(NewStanValue6[,33])+as.numeric(NewStanValue6[,36]),digits=2) #Intimacy
NewMatrix5[,4]<-round(as.numeric(NewStanValue6[,14])+as.numeric(NewStanValue6[,16])+as.numeric(NewStanValue6[,22])+as.numeric(NewStanValue6[,27])+as.numeric(NewStanValue6[,29])+as.numeric(NewStanValue6[,30])+as.numeric(NewStanValue6[,31])+as.numeric(NewStanValue6[,32])+as.numeric(NewStanValue6[,35])+as.numeric(NewStanValue6[,37])+as.numeric(NewStanValue6[,38]),digits=2) #Cognitive Simplicity
#head(NewMatrix5)
#Step 4: Set up matrix of the variable 'populism' but grouping all the sub-sub variables
NewMatrix6<-array(NA,dim=c(71,2))
NewMatrix6[,1]<-StanValue6[,1]#names of year are the first column
for(j in 1:71)#row loop
{
rowsum=0
for(i in 2:(dictnumber+1))#column loop
{
rowsum<-rowsum+as.numeric(NewStanValue6[j,i])
}#end column loop
NewMatrix6[j,2]<-round(rowsum,digits=2)
}#end row loop
#NewMatrix6
#Step 5: Compare with the uncorrected set
NewMatrix7<-array(NA,dim=c(71,2))
NewMatrix7[,1]<-StanValue6[,1]#names of year are the first column
for(j in 1:71)#row loop
{
rowsum=0
for(i in 2:(dictnumber+1))#column loop
{
rowsum<-rowsum+as.numeric(StanValue6[j,i])
}#end column loop
NewMatrix7[j,2]<-round(rowsum,digits=2)
}#end row loop
NewMatrix7
Anova 2
#For the F values of the sub variables
NewMatrix3z <- cbind(NewMatrix3,popdicodfmHspeechWeighttwolevels@docvars$loc)
colnames(NewMatrix3z)<-c("Speech","Deintermediation","Intimacy","Simplicity","PM")
resultanova5 <- aov(as.matrix(Deintermediation)~as.factor(PM), data=as.data.frame(NewMatrix3z))
resultanova6 <- aov(as.matrix(Intimacy)~as.factor(PM), data=as.data.frame(NewMatrix3z))
resultanova7 <- aov(as.matrix(Simplicity)~as.factor(PM), data=as.data.frame(NewMatrix3z))
fvaluelist5<-round(summary.aov(resultanova5)[[1]][["F value"]][[1]],digits=2)
fvaluelist6<-round(summary.aov(resultanova6)[[1]][["F value"]][[1]],digits=2)
fvaluelist7<-round(summary.aov(resultanova7)[[1]][["F value"]][[1]],digits=2)
resultanovapm5<-eta_sq(resultanova5)
resultanovapm5$etasq<-round(resultanovapm5$etasq,digits=2)
resultanovapm6<-eta_sq(resultanova6)
resultanovapm6$etasq<-round(resultanovapm6$etasq,digits=2)
resultanovapm7<-eta_sq(resultanova7)
resultanovapm7$etasq<-round(resultanovapm7$etasq,digits=2)
fvaluesfordf5<-c("F",fvaluelist5,fvaluelist6,fvaluelist7)
anovaresultsfordf5<-c("n^2",resultanovapm5$etasq,resultanovapm6$etasq,resultanovapm7$etasq)
Table2final<-rbind(NewMatrix,fvaluesfordf5,anovaresultsfordf5)
Table2final
colnames(Table2final)<-c("Prime Minister","Deintermediation","Intimacy","Simplicity")
Table2finalDF<-as.data.frame(Table2final,row.names=FALSE)
Table2finalDF
#For the F values of the populism variable
NewMatrix4z <- cbind(NewMatrix4,popdicodfmHspeechWeightonelevel@docvars$loc)
colnames(NewMatrix4z)<-c("Speech","Populism","PM")
resultanova8 <- aov(as.matrix(Populism)~as.factor(PM), data=as.data.frame(NewMatrix4z))
fvaluelist8<-round(summary.aov(resultanova8)[[1]][["F value"]][[1]],digits=2)
resultanovapm8<-eta_sq(resultanova8)
resultanovapm8$etasq<-round(resultanovapm8$etasq,digits=2)
fvaluesfordf8<-c("F",fvaluelist8)
anovaresultsfordf8<-c("n^2",resultanovapm8$etasq)
Table3my<-rbind(NewMatrix2,fvaluesfordf8,anovaresultsfordf8)
colnames(Table3my)<-c("Prime Minister","Tested Dictionary")
Table3final <- cbind(Table3my,Table4[,2])
colnames(Table3final)<-c("Prime Minister","Tested Dictionary","Pauwel's Dictionary")
Table3finalDF<-as.data.frame(Table3final,row.names=FALSE)
Table3finalDF
#Table3final<-rbind(StanValue3,fvaluesfordf8,anovaresultsfordf8)
#Table3final
#colnames(Table3final)[1]<-"Prime Minister"
#pm8 <- as.factor(popdicodfmHspeechWeighttwolevels@docvars$loc)
#NewMatrixt3v <- gsub(".txt", "", NewMatrix3)
#NewMatrix3DF <- as.data.frame(NewMatrix3)
#NewMatrixt3t <- gsub(".txt", "", NewMatrix3)
#NewMatrix3DF
#popdicodfmHspeechWeighttwolevels
#NewMatrix3z <- cbind(NewMatrix3,popdicodfmHspeechWeighttwolevels@docvars$loc)
#NewMatrix3z
#colnames(NewMatrix3z)<-c("Speech","Deintermediation","Intimacy","Simplicity","PM")
#NewMatrix3bis <- NewMatrix3[,-1]
#rownames(NewMatrix3bis) <- NewMatrix3[,1]
#NewMatrix3t
#length(pm8)
#NewMatrixt <- gsub(".txt", "", NewMatrix)
Post-analysis checks
#resultanova$coefficients
#anova(resultanova)
#Step 2: Post-hoc Tukey
#First all the anovas of the sub-sub-variables have to be calculated separately
#populist.intimacy.family
popdicodfmHspeechWeightasdf <- convert(popdicodfmHspeechWeight, to = "data.frame")
resultanovafamily1 = aov(popdicodfmHspeechWeightasdf$family~as.factor(pm))
resultanovafamily1
resultanovafamily2 = aov(popdicodfmHspeechWeightasdf[,12]~as.factor(pm))
resultanovafamily2
summary.aov(resultanovafamily1)
#populist.intimacy.emotion
#!!!!! DO THAT WITH THE OTHER SUB-SUB-VARIABLES OF THE DICTIONARY WHEN FINALISED !!!!!
#Then we do group-wise comparisons with Tukey test
TukeyHSD(aov(resultanovafamily1))
#Step 3: Post-hoc Bonferroni, first the p-values of each sub-sub-variable are reported into the pv object
pv<-c(2.2e-16) #nb: p-values of several sub-sub-variables can be added up, e.g. pv<-c(2.2e-17,3.7e-6,4.8e-3)
bonferroniadjust <- p.adjust(pv, method = "bonferroni", n = 11) #should be no of speakers
bonferroniadjust
#Step 4: Compute conservative effect sizes
eta_sq(resultanova) #check once the parameters
eta_sq(resultanovafamily1)
#PART 2: With the sub-variables of the dictionary of the article (weighted, 2 levels)
#Step 1: Anova
pm2 <- as.factor(popdicodfmHspeechWeighttwolevels@docvars$loc)
head(pm2)
resultanova2 = aov(as.matrix(popdicodfmHspeechWeighttwolevels)~as.factor(pm2)) #check caps & regex once
summary.aov(resultanova2)
resultanova2$coefficients
#anova(resultanova2)
#Step 2: Post-hoc Tukey
#First all the anovas of the sub-variables have to be calculated separately
#Populism.Intimacy
popdicodfmHspeechWeightasdf <- convert(popdicodfmHspeechWeighttwolevels, to = "data.frame")
resultanovadeintermediation = aov(popdicodfmHspeechWeightasdf$Deintermediation~as.factor(pm2))
summary.aov(resultanovafamily1)
#populist.intimacy.emotion
#!!!!! DO THAT WITH THE OTHER SUB-SUB-VARIABLES OF THE DICTIONARY WHEN FINALISED !!!!!
#Then we do group-wise comparisons with Tukey test
TukeyHSD(aov(resultanovadeintermediation))
#Step 3: Post-hoc BonferronI, the p-values of each sub-variable are printed into the pv object
pv<-c(2.2e-16) #nb: p-values of several sub-sub-variables can be added up, e.g. pv<-c(2.2e-17,3.7e-6,4.8e-3)
bonferroniadjust <- p.adjust(pv, method = "bonferroni", n = 11) #should be no of speakers
bonferroniadjust
#Step 4: Compute conservative effect sizes
eta_sq(resultanova2) #check once the parameters
eta_sq(resultanovadeintermediation)
#PART 3:With the entire dictionary of the article (weighted, 1 level)
#Step 1: Anova
pm3 <- as.factor(popdicodfmHspeechWeightonelevel@docvars$loc)
head(pm3)
resultanova3 = aov(as.matrix(popdicodfmHspeechWeightonelevel)~as.factor(pm3)) #check caps & regex once
summary.aov(resultanova3)
resultanova3$coefficients
#anova(resultanova3)
#Step 2: Post-hoc Tukey, a group-wise comparisons with Tukey test is performed
TukeyHSD(aov(resultanova3))
#Step 3: Post-hoc Bonferroni
#First we report the p-values of each sub-sub-variable into the pv object
pv<-c(2.2e-16) #nb: p-values of several sub-sub-variables can be added up, e.g. pv<-c(2.2e-17,3.7e-6,4.8e-3)
bonferroniadjust <- p.adjust(pv, method = "bonferroni", n = 11) #should be no of speakers
bonferroniadjust
#Step 4: Compute conservative effect sizes
eta_sq(resultanova3) #check once the parameters
#PART 4:With the dictionary of Pauwels (2011) (weighted, 1 level)
#Step 1: Anova
pm4 <- as.factor(popdicodfmLspeechWeightonelevel@docvars$loc)
head(pm4)
resultanova4 = aov(as.matrix(popdicodfmLspeechWeightonelevel)~as.factor(pm4)) #check caps & regex once
summary.aov(resultanova4)
resultanova4$coefficients
#anova(resultanova3)
#Step 2: Post-hoc Tukey, a group-wise comparisons with Tukey test is performed
TukeyHSD(aov(resultanova4))
#Step 3: Post-hoc Bonferroni
#First we report the p-values of each sub-sub-variable into the pv object
pv<-c(2.2e-16) #nb: p-values of several sub-sub-variables can be added up, e.g. pv<-c(2.2e-17,3.7e-6,4.8e-3)
bonferroniadjust <- p.adjust(pv, method = "bonferroni", n = 11) #should be no of speakers
bonferroniadjust
#Step 4: Compute conservative effect sizes
eta_sq(resultanova4) #check once the parameters
#BONUS PART: plot results (weighted, two levels, one list of words)
#ggplot(popdicodfmHspeechWeightasdf, aes(x = as.factor(pm2), y = popdicodfmHspeechWeightasdf$family)) +
#geom_boxplot(fill = "grey80", colour = "black") +
#scale_x_discrete() + xlab("Prime Ministers") +
#ylab("Family vocabulary")
Generalized Linear Model
Here the results of the anova are put into perspecive using a countinous variable (time). For more on the commands, c.f. https://r-statistics.co/Linear-Regression.html.
#Step 1: Preparation of the dictionaries to fit a timewise analysis
#weighted dictionaries (yearwise and timewise)
dfmperyearw <- dfm_weight(dfmperyear,"prop")
dfmperdayw <- dfm_weight(dfmperday,"prop")
#popdicodfmHpmYWeight is the weighted dictionary matrix if corpus is organised yearwise (3 levels)
popdicodfmHpmYWeight <- dfm_lookup(dfmperyear, dictionary = popdicoH, valuetype = "glob", levels=3)
#popdicodfmHpmDWeight is the weighted dictionary matrix if corpus is organised daywise
popdicodfmHpmDWeight <- dfm_lookup(dfmperdayw, dictionary = popdicoH, valuetype = "glob", levels=3)
#popdicodfmHpmYWeighttwolevels is the weighted dictionary matrix if corpus is organised yearwise (2 levels)
popdicodfmHpmYWeighttwolevels <- dfm_lookup(dfmperyear, dictionary = popdicoH, valuetype = "glob", levels=2)
#popdicodfmHpmDWeighttwolevels is the weighted dictionary matrix if corpus is organised daywise
popdicodfmHpmDWeighttwolevels <- dfm_lookup(dfmperdayw, dictionary = popdicoH, valuetype = "glob", levels=2)
#popdicodfmHpmYWeightonelevel is the weighted dictionary matrix if corpus is organised yearwise (1 level)
popdicodfmHpmYWeightonelevel <- dfm_lookup(dfmperyear, dictionary = popdicoH, valuetype = "glob", levels=1)
#popdicodfmHpmDWeightonelevel is the weighted dictionary matrix if corpus is organised daywise
popdicodfmHpmDWeightonelevel <- dfm_lookup(dfmperdayw, dictionary = popdicoH, valuetype = "glob", levels=1)
#popdicodfmHpmYWeightonelevel is the weighted dictionary matrix if corpus is organised yearwise (1 level) Pauwels
popdicodfmLpmYWeightonelevel <- dfm_lookup(dfmperyear, dictionary = popdicoL, valuetype = "glob", levels=1)
#popdicodfmHpmDWeightonelevel is the weighted dictionary matrix if corpus is organised daywise (1 level) Pauwels
popdicodfmLpmDWeightonelevel <- dfm_lookup(dfmperdayw, dictionary = popdicoL, valuetype = "glob", levels=1)
#Step 2: Linear Regression (yearwise, weighted, 3 levels)
#General Analysis (all the dictionary lists at once)
pm5 <- as.factor(popdicodfmHspeechWeight@docvars$year)
head(pm5)
resultlm5 <- lm(as.matrix(popdicodfmHspeechWeight)~as.factor(pm5)) #check caps & regex once #print(resultlm5)
#summary(resultlm5) #takes time! #resultlm5$coefficients
#Analysis per sub-sub populist variable (e.g. family)
popdicodfmHpmYWeightdf <- convert(popdicodfmHpmYWeight, to = "data.frame")
resultlmFamily <- lm(popdicodfmHpmYWeightdf$family~popdicodfmHpmYWeight@docvars$year)
summary(resultlmFamily)
resultlmFamily$coefficients
AIC(resultlmFamily)
BIC(resultlmFamily)
summary(resultlmFamily)$r.squared
logLik(resultlmFamily)
dfmperyearwasdf <- convert(dfmperyearw, to = "data.frame")
scatter.smooth(x=dfmperyearwasdf$document, y=popdicodfmHpmYWeightdf$family, main="Year ~ Family") #plot results
#!!!!! DO THAT WITH THE OTHER SUB-SUB-VARIABLES OF THE DICTIONARY WHEN FINALISED !!!!!
#Step 3: Linear Regression (yearwise, weighted, 1 level, article's dictionary)
#General Analysis
pm6 <- as.factor(popdicodfmHspeechWeightonelevel@docvars$year)
head(pm6)
resultlm6 = lm(as.matrix(popdicodfmHspeechWeightonelevel)~as.factor(pm6)) #check caps & regex once
#summary(resultlm6)
#resultlm6$coefficients
AIC(resultlm6)
BIC(resultlm6)
summary(resultlm6)$r.squared
logLik(resultlm6)
dfmperyearwasdf <- convert(dfmperyearw, to = "data.frame")
scatter.smooth(x=dfmperyearwasdf$document, y=popdicodfmHpmYWeightonelevel, main="Year ~ Populism")
#Step 4: Linear Regression (yearwise, weighted, 1 level, Pauwels dictionary)
#General Analysis
pm7 <- as.factor(popdicodfmLspeechWeightonelevel@docvars$year)
head(pm7)
resultlm7 = lm(as.matrix(popdicodfmLspeechWeightonelevel)~as.factor(pm7)) #check caps & regex once
#summary(resultlm7)
#resultlm6$coefficients
AIC(resultlm7)
BIC(resultlm7)
summary(resultlm7)$r.squared
logLik(resultlm7)
dfmperyearwasdf <- convert(dfmperyearw, to = "data.frame")
scatter.smooth(x=dfmperyearwasdf$document, y=popdicodfmLpmYWeightonelevel, main="Year ~ Populism")
#Replication of step 3: linear regression with quadratic (square) term with quadratic term scatterplot
a <- as.matrix(popdicodfmHpmYWeightonelevel)
a <- cbind(a, as.numeric(as.character(rownames(a))))
a <- as.data.frame(a)
colnames(a) <- c("Populism", "Year")
plot(a$Year, a$Populism)
a$Year2 <- a$Year^2
r1 <- lm(a$Populism ~ a$Year + a$Year2)
scatterplot( a$Populism~ a$Year, data=a, xlab="Year", ylab="Populism", main="Year ~ Populism", xlim=c( 1946,2019 ), col="black" )
lines(a$Year,predict(r1), col = "red")
#linear regression with quadratic (square) term withough scatterplot
#a$Year2 <- a$Year^2
#r1 <- lm(a$Populism ~ a$Year + a$Year2)
#plot(a$Year, a$Populism)
#lines(a$Year, predict(r1), col = "black")
#replication of step 3 using glm instrad of lm:
#lm.ts <- glm(a$Populism ~ a$Year + a$Year2)
#summary( lm.ts )
#durbinWatsonTest( lm.ts, max.lag = 6 )
#jarque.bera.test( resid( lm.ts ))
#anova( lm.ts )
#Using popdicodfmHspeechWeightonelevel instead of popdicodfmHpmYWeightonelevel
#a2 <- as.matrix(popdicodfmHspeechWeightonelevel)
#a2 <- as.data.frame(cbind(a2, as.numeric(as.character(pm6))))
#colnames(a2) <- c("Populism", "Year_v1")
#a2$Year_v2 <- substr(rownames(a2), 2,5)
#check <- a2[(a2$Year_v1 != a2$Year_v2),]
#a2 <- aggregate(Populism ~ Year_v2, data = a2, FUN = "mean")
#plot(a2$Year, a2$Populism)
#plot(a$Populism, a2$Populism)
New Generalized Linear Model
The standard deviation of the mean for the populist dictionary is plotted year-wise.
#year-wise
NewMatrix6DF<-as.data.frame(NewMatrix6)
NewMatrix7DF<-as.data.frame(NewMatrix7)
y <- as.matrix(NewMatrix6) #NewMatrix6 or #NewMatrix7
y <- cbind(y, as.numeric(as.character(rownames(y))))
y <- as.data.frame(y)
colnames(y) <- c("Year", "Populism")
y$Populism <- as.numeric(as.character(y$Populism)) #To treat populism as a numeric variable
#plot(y$Year,y$Populism,pch=4) #if year treated as character
plot(as.numeric(as.character(y$Year)),y$Populism, pch=4) #if year treated as numeric ####FIGURE 3A
resultlm7 = lm(y$Populism~ as.numeric(y$Year),data=y)
summary(resultlm7)
#myts <- ts(y$Year, start=c(1946, 1), end=c(2019, 1), frequency=1)
y$Year2 <- (as.numeric(y$Year^2))
r1 <- lm(y$Populism ~ y$Year)
scatterplot( y$Populism~ y$Year, data=y, xlab="Year", ylab="Populism", main="Year ~ Populism", xlim=c( 1946,2019 ), col="black" )
lines(y$Year,predict(r1), col = "red")
summary(resultlm7)
resultlm7$coefficients
AIC(resultlm7)
BIC(resultlm7)
summary(resultlm7)$r.squared
logLik(resultlm7)
#speach-wise
NewMatrix4DF<-as.data.frame(NewMatrix4)
s <- as.matrix(NewMatrix4)
s <- cbind(s, as.numeric(as.character(rownames(s))))
s <- as.data.frame(s)
colnames(s) <- c("Speech", "Populism")
s$Populism <- as.numeric(as.character(s$Populism)) #To treat populism as a numeric variable
plot(s$Speech,s$Populism) #if year treated as character
#plot(as.numeric(y$Year),y$Populism) #if year treated as numeric
resultlm8 = lm(s$Populism~ as.numeric(s$Speech),data=s)
summary(resultlm8)
#add other variables to the speech-wise dictionary
z <- cbind(s,popdicodfmHspeechWeightonelevel@docvars$loc,popdicodfmHspeechWeightonelevel@docvars$year,popdicodfmHspeechWeightonelevel@docvars$month,popdicodfmHspeechWeightonelevel@docvars$day,popdicodfmHspeechWeightonelevel@docvars$period,popdicodfmHspeechWeightonelevel@docvars$term,popdicodfmHspeechWeightonelevel@docvars$govt,popdicodfmHspeechWeightonelevel@docvars$typegeneral,popdicodfmHspeechWeightonelevel@docvars$format,popdicodfmHspeechWeightonelevel@docvars$independence,popdicodfmHspeechWeightonelevel@docvars$independencerepublicday,popdicodfmHspeechWeightonelevel@docvars$country,popdicodfmHspeechWeightonelevel@docvars$state,popdicodfmHspeechWeightonelevel@docvars$city,popdicodfmHspeechWeightonelevel@docvars$area,popdicodfmHspeechWeightonelevel@docvars$language,popdicodfmHspeechWeightonelevel@docvars$pmyear,popdicodfmHspeechWeightonelevel@docvars$no)
colnames(z) <- c("Speech", "Populism","PM","Year","Month","Day","Period","Term","Govt","Type","Format","Ind","IndRep","Country","State","City","Area","Language","PMyear","No")
z <- as.data.frame(z)
z$Populism <- as.numeric(as.character(z$Populism))
plot(z$PM,z$Populism) ########## FIGURE 2
hist(z$Populism)
plot(density(z$Populism))
nll.normal <-function(data,par){
return(-sum(log(dnorm(data, mean=par[1], sd=par[2]))))
}
#optim(par=c(1,0.1), fn=nll.normal, data=z$Populism)
z$Year2 <- z$Year^2
r2 <- lm(z$Populism ~ z$Year + z$Year2)
scatterplot( z$Populism~ z$Year, data=z, xlab="Year", ylab="Populism", main="Year ~ Populism", xlim=c(1946,2019 ), col="black" )
lines(z$Year,predict(r2), col = "red")
resultlm9 = lm(z$Populism~ as.numeric(z$Year),data=z)
summary(resultlm9)
summary(resultlm9)
resultlm9$coefficients
AIC(resultlm9)
BIC(resultlm9)
summary(resultlm9)$r.squared
logLik(resultlm9)
#add other variables to the speech-wise dictionary with all the sub sub variables
colnames(NewStanValue5)<-ColumnNames
colnames(StanValue5)<-ColumnNames
remove(q)
q <- as.matrix(StanValue5) #NewStanValue5 if inverse relations, #StanValue5 with renamed columned #StanValue #popdicodfmHspeechWeight otherwise
r <- cbind(popdicodfmHspeechWeight@docvars$year,popdicodfmHspeechWeight@docvars$loc,q)
colnames(r)[1]<-"Year"
colnames(r)[2]<-"PM"
r<-as.data.frame(r)
Time <- time(as.numeric(r$Year)) #This is necessary to consider r$Year as a time series
#r$Year <- as.numeric(z$Year)
#r$PM <- as.numeric(z$PM)
r$institutionalprocesses <- as.numeric(as.character(r$institutionalprocesses))
r$politicalparties<- as.numeric(as.character(r$politicalparties))
r$firstpersonsingular<- as.numeric(as.character(r$firstpersonsingular))
r$firstpersonplural<- as.numeric(as.character(r$firstpersonplural))
r$thirdpersonsingular<- as.numeric(as.character(r$thirdpersonsingular))
r$thirdpersonplural<- as.numeric(as.character(r$thirdpersonplural))
r$risk<- as.numeric(as.character(r$risk))
r$assent<- as.numeric(as.character(r$assent))
r$electoralprocesses<- as.numeric(as.character(r$electoralprocesses))
r$personalisedgovernance<- as.numeric(as.character(r$personalisedgovernance))
r$family<- as.numeric(as.character(r$family))
r$interrogatives<- as.numeric(as.character(r$interrogatives))
r$rhetoricalquestion<- as.numeric(as.character(r$rhetoricalquestion))
r$religion<- as.numeric(as.character(r$religion))
r$communities<- as.numeric(as.character(r$communities))
r$emotionaltone<- as.numeric(as.character(r$emotionaltone))
r$positiveemotions<- as.numeric(as.character(r$positiveemotions))
r$negativeemotions<- as.numeric(as.character(r$negativeemotions))
r$malereferences<- as.numeric(as.character(r$malereferences))
r$femalereferences<- as.numeric(as.character(r$femalereferences))
r$festival<- as.numeric(as.character(r$festival))
r$leisure<- as.numeric(as.character(r$leisure))
r$body<- as.numeric(as.character(r$body))
r$health <- as.numeric(as.character(r$health))
r$friends<- as.numeric(as.character(r$friends))
r$nonelite<- as.numeric(as.character(r$nonelite))
r$home<- as.numeric(as.character(r$home))
r$simplewords<- as.numeric(as.character(r$simplewords))
r$shortsentences<- as.numeric(as.character(r$shortsentences))
r$numbers<- as.numeric(as.character(r$numbers))
r$cognitiveprocesses<- as.numeric(as.character(r$cognitiveprocesses))
r$motion<- as.numeric(as.character(r$motion))
r$pastfocus<- as.numeric(as.character(r$pastfocus))
r$certainty<- as.numeric(as.character(r$certainty))
r$time<- as.numeric(as.character(r$time))
r$festivalandculturalrefs<- as.numeric(as.character(r$festivalandculturalrefs))
r$conceptualnotions<- as.numeric(as.character(r$conceptualnotions))
dependentvars <- cbind(r$institutionalprocesses+r$politicalparties+r$firstpersonsingular+r$firstpersonplural+r$thirdpersonsingular+r$thirdpersonplural+r$risk+r$assent+r$electoralprocesses+r$personalisedgovernance+r$family+r$interrogatives+r$rhetoricalquestion+r$religion+r$communities+r$emotionaltone+r$positiveemotions+r$negativeemotions+r$malereferences+r$femalereferences+r$festival+r$leisure+r$body+r$health+r$friends+r$nonelite+r$home+r$simplewords+r$shortsentences+r$numbers+r$cognitiveprocesses+r$motion+r$pastfocus+r$certainty+r$time+r$festivalandculturalrefs+r$conceptualnotions)
#r3 <- lm(r$family & r$institutionalprocesses ~ Time)
r3 <- lm(cbind(r$institutionalprocesses,r$politicalparties,r$firstpersonsingular,r$firstpersonplural,r$thirdpersonsingular,r$thirdpersonplural,r$risk,r$assent,r$electoralprocesses,r$personalisedgovernance,r$family,r$interrogatives,r$rhetoricalquestion,r$religion,r$communities,r$emotionaltone,r$positiveemotions,r$negativeemotions,r$malereferences,r$femalereferences,r$festival,r$leisure,r$body,r$health,r$friends,r$nonelite,r$home,r$simplewords,r$shortsentences,r$numbers,r$cognitiveprocesses,r$motion,r$pastfocus,r$certainty,r$time,r$festivalandculturalrefs,r$conceptualnotions) ~ Time, data=r)
r4 <- lm(dependentvars ~ Time, data=r)
summary(r3)
summary(r4)
#try with GLM
lm.ts <- glm( Time ~ r$institutionalprocesses+r$politicalparties+r$firstpersonsingular+r$firstpersonplural+r$thirdpersonsingular+r$thirdpersonplural+r$risk+r$assent+r$electoralprocesses+r$personalisedgovernance+r$family+r$interrogatives+r$rhetoricalquestion+r$religion+r$communities+r$emotionaltone+r$positiveemotions+r$negativeemotions+r$malereferences+r$femalereferences+r$festival+r$leisure+r$body+r$health+r$friends+r$nonelite+r$home+r$simplewords+r$shortsentences+r$numbers+r$cognitiveprocesses+r$motion+r$pastfocus+r$certainty+r$time+r$festivalandculturalrefs+r$conceptualnotions, data=r) #r$motion == r$emotionaltone & r$malereferences == r$femalereferences & r$festivalandculturalrefs == r$festival are perfectly collinear
summary( lm.ts )
alias(lm.ts)
durbinWatsonTest( lm.ts, max.lag = 6 )
jarque.bera.test( resid( lm.ts ))
anova( lm.ts )
lm.ts <- glm( Time ~ r$institutionalprocesses+r$politicalparties+r$firstpersonsingular+r$firstpersonplural+r$thirdpersonsingular+r$thirdpersonplural+r$risk+r$assent+r$electoralprocesses+r$personalisedgovernance+r$family+r$interrogatives+r$rhetoricalquestion+r$religion+r$communities+r$emotionaltone+r$positiveemotions+r$negativeemotions+r$malereferences+r$leisure+r$body+r$health+r$friends+r$nonelite+r$home+r$simplewords+r$shortsentences+r$numbers+r$cognitiveprocesses+r$pastfocus+r$certainty+r$time+r$festivalandculturalrefs+r$conceptualnotions, data=r) #collinear variables dropped
#plot(as.numeric(as.character(y$Year), as.numeric(as.character(y$Populism))))
#> plot(y$Year,y$Populism)
#plot(as.numeric(as.character(y$Year), as.numeric(as.character(y$Populism))))
#> scatterplot(y$Populism~ y$Year, data=y)
#> plot(as.numeric(y$Year),y$Populism)
#> y$Populism <- as.numeric(as.character(y$Populism))
plot(as.numeric(y$Year),y$Populism)
#scatterplot(y$Populism~ y$Year, data=y)
#y$Year2 <- y$Year^2
#r1 <- lm(y$Populism ~ y$Year + y$Year2)
#scatterplot( y$Populism~ y$Year, data=y, xlab="Year", ylab="Populism", main="Year ~ Populism", xlim=c( 1946,2019 ), col="black" )
#lines(a$Year,predict(r1), col = "red")
Factor Analysis
#from Chris
n <- 100
theta <- rnorm(n,0,1)
alpha1 <- -1.000000
beta1 <- 1.000000
alpha2 <- 0.000000
beta2 <- 1.000000
alpha3 <- 1.000000
beta3 <- 3.000000
k <- 3
x1 <- alpha1 + beta1 * theta + rnorm(n)
x2 <- alpha2 + beta2 * theta + rnorm(n)
x3 <- alpha3 + beta3 * theta + rnorm(n)
x <- cbind(x1, x2, x3)
fit <- factanal(x, factor=1, scores="regression")
par(mar=c(4,4,1,1), font=2, font.lab=2, cex=1.3)
plot(fit$scores, theta, xlim=c(-3,3), ylim=c(-3,3), ylab="true theta", xlab="factor analysis scores")
abline(a=0, b=1, col=2, lwd=2)
#Cbinding the various dictionary lists
fa <- cbind(r$institutionalprocesses,r$politicalparties,r$firstpersonsingular,r$firstpersonplural,r$thirdpersonsingular,r$thirdpersonplural,r$risk,r$assent,r$electoralprocesses,r$personalisedgovernance,r$family,r$interrogatives,r$rhetoricalquestion,r$religion,r$communities,r$emotionaltone,r$positiveemotions,r$negativeemotions,r$malereferences,r$leisure+r$body,r$health+r$friends,r$nonelite,r$home,r$simplewords,r$shortsentences,r$numbers,r$cognitiveprocesses,r$pastfocus,r$certainty,r$time,r$festivalandculturalrefs,r$conceptualnotions) #collinear variables dropped
#PCA to determine the number of useful factors
poppca <- princomp(fa)
summary(poppca)
plot(poppca)
#Another method to determine the number of useful factors
library(nFactors)
ev <- eigen(cor(fa))
ap <- parallel(subject=nrow(fa),var=ncol(fa),
rep=100,cent=.05)
nS <- nScree(x=ev$values, aparallel=ap$eigen$qevpea)
plotnScree(nS)
#Exploratory Factor analysis using x factors
fit <- factanal(fa, factor=2, scores="regression")
summary(fit)
print(fit, digits=2, cutoff=.3, sort=TRUE)
head(fit$scores)
#Confirmatory Factor analysis
library(lavaan)
colnames(NewStanValue5)<-ColumnNames
vector <- as.vector(na.omit(as.character(r$institutionalprocesses)))
vector2 <- as.vector(na.omit(as.character(r$politicalparties)))
model0 <- 'Deintermediation =~ vector + vector2'
model1 <- ' Deintermediation =~ as.factor(r$institutionalprocesses)+ as.factor(r$politicalparties)'
model1 <- ' Deintermediation =~ r$institutionalprocesses+ r$politicalparties+ r$firstpersonsingular+ r$firstpersonplural+ r$thirdpersonsingular+ r$thirdpersonplural+ r$electoralprocesses+ r$personalisedgovernance+ r$pastfocus
Intimacy =~ r$risk+ r$assentr$family+ r$interrogatives+ r$religion+ r$communities+ r$emotionaltone+ r$positiveemotions+ r$negativeemotions+ r$malereferences+ r$leisure+ r$body+ r$health+ r$friends+ r$home+ r$time
Simplicity =~ r$rhetoricalquestion+ r$nonelite+ r$simplewords+ r$shortsentences+ r$numbers+ r$cognitiveprocesses+ r$certainty+ r$festivalandculturalrefs+ r$conceptualnotions'
#fit2 <- cfa(model0, data=r, missing="default")
#Deintermediation
#r$institutionalprocesses+r$politicalparties+r$firstpersonsingular+r$firstpersonplural+r$thirdpersonsingular+r$thirdpersonplural+r$electoralprocesses+r$personalisedgovernance+r$pastfocus
#Intimacy
#r$risk+r$assentr$family+r$interrogatives+r$religion+r$communities+r$emotionaltone+r$positiveemotions+r$negativeemotions+r$malereferences+r$leisure+r$body+r$health+r$friends+r$home+r$time
#Cognitive simplicity
#r$rhetoricalquestion+r$nonelite+r$simplewords+r$shortsentences+r$numbers+r$cognitiveprocesses+r$certainty+r$festivalandculturalrefs+r$conceptualnotions
?lavaan
Proportions instead of mean standard deviations
#Pre-part 1, checking stuff by removing the x1000 indicator
for(i in 2:38){
if(i==2){plot(as.numeric(as.character(popdicodfmHpmWeightDF[,i]))/1000,type="l",ylim=c(0,0.1))}else{lines(as.numeric(as.character(popdicodfmHpmWeightDF[,i]))/1000,col=i)}
}
boxplot(as.numeric(as.character(popdicodfmHpmWeightDF[,2]))/1000)
#Pre-part 2, checking that the sum is 1
test <- dfm_weight(popdicodfmHpm,"prop")
apply(test, 1, sum)
#Part 1: add a column with the rest of the corpus
#weightdfmtokssubworkcorpus<-dfm_weight(dfmtokssubworkcorpus,"prop")
popdicodfmHspeechWeight <- dfm_lookup(weightdfmtokssubworkcorpus, dictionary = popdicoH, valuetype = "glob", levels=3)
nostoptokssubworkcorpus <- tokens_select(tokssubworkcorpus, pattern = popdicoH, selection = 'remove')
keeponlydico <- tokens_select(ngramstokssubworkcorpus, pattern = popdicoH, valuetype = 'glob', case_insensitive = FALSE, selection = 'keep')
dfmkeeponlydico <- dfm(keeponlydico, remove_punct = FALSE, tolower = FALSE, dictionary_regex=TRUE, language = "english", stem = FALSE, clean = FALSE, verbose= TRUE)
onlytherest <- tokens_select(ngramstokssubworkcorpus, pattern = popdicoH, valuetype = 'glob', case_insensitive = FALSE, selection = 'remove')
dfmonlytherest <- dfm(onlytherest, remove_punct = FALSE, tolower = FALSE, dictionary_regex=TRUE, language = "english", stem = FALSE, clean = FALSE, verbose= TRUE)
catdfmkeeponlydico <- dfm_lookup(dfmkeeponlydico, dictionary = popdicoH, levels=3)
u <-dfmonlytherest
U<-rowSums(u)
#U
#head(U)
#dim(U)
#dfmtokssubworkcorpus@docvars$loc,dfmtokssubworkcorpus@docvars$year
#dfmtokssubworkcorpus@docvars$loc
#dfmtokssubworkcorpus@docvars$year
kcsdmk <- cbind(as.character(dfmtokssubworkcorpus@docvars$loc),dfmtokssubworkcorpus@docvars$year)
v <- cbind(catdfmkeeponlydico,U)
v2<-convert(cbind(dfmtokssubworkcorpus@docvars$year,v),to="data.frame")
v3<-cbind(dfmtokssubworkcorpus@docvars$loc,v2)
colnames(v3)[1]<-"PM"
colnames(v3)[2]<-"Speech"
colnames(v3)[3]<-"Year"
#Part 2: Computing the percentage of each column
v4 <- v3
for(i in 1:nrow(v3)){
for(j in 4:ncol(v3)){
v4[i,j]<-v3[i,j]/sum(v3[i,(4:ncol(v3))]) #words per speech matlab... sum(v3[i,(4:ncol(v3) | each cell...v3[i,j]
}
}
#Part 3: Removing the few empty texts
v5<-apply(v4[,-(1:3)], 1, sum)
print(v4[117,])
#v5
which(v5=="NaN")
v4<-v4[-3601,]
v4<-v4[-3579,]
v4<-v4[-1582,]
v4<-v4[-150,]
v4<-v4[-117,]
#Part 4: Grouping variables in 3 groups (well, 4 now but 3 soon)
colnames(v4)[1]<-"PM"
colnames(v4)[2]<-"Speech"
colnames(v4)[3]<-"Year"
populism <- cbind(v4$firstpersonsingular+v4$thirdpersonsingular+v4$electoralprocesses+v4$personalisedgovernance+v4$family+v4$rhetoricalquestion+v4$religion+v4$communities+v4$emotionaltone+v4$positiveemotions+v4$negativeemotions+v4$malereferences+v4$femalereferences+v4$festival+v4$leisure+v4$body+v4$health+v4$friends+v4$nonelite+v4$home+v4$shortsentences+v4$numbers+v4$cognitiveprocesses+v4$pastfocus+v4$time+v4$festivalandculturalrefs)
nonpopulism <- cbind(v4$institutionalprocesses+v4$politicalparties+v4$firstpersonplural+v4$thirdpersonplural+v4$risk+v4$assent+v4$interrogatives+v4$motion+v4$certainty+v4$conceptualnotions)
neutral <- cbind(v4$feat11)
shortwords <- cbind(v4$simplewords)
PM <- cbind(v4$PM)
Year <- cbind(v4$Year)
#Part 5: Plotting results
scatterplot(Year, populism)
plot(v4$PM, populism, main="Populism", sub="(stylistic features)",
xlab="Prime Ministers", ylab="% of tokens")
plot(v4$PM, nonpopulism, main="Populist averse", sub="(stylistic features)",
xlab="Prime Ministers", ylab="% of tokens")
plot(v4$PM, neutral, main="Neutral", sub="(stylistic features)",
xlab="Prime Ministers", ylab="% of tokens")
plot(v4$PM, shortwords, main="Shortwords", sub="(stylistic features)",
xlab="Prime Ministers", ylab="% of tokens")
#Part 6: Anova
#1 Grouping variables of interest and naming them (all the vars)
allvars <- cbind(v4$institutionalprocesses,v4$politicalparties,v4$firstpersonsingular,v4$firstpersonplural,v4$thirdpersonsingular,v4$thirdpersonplural,v4$risk,v4$assent,v4$electoralprocesses,v4$personalisedgovernance,v4$family,v4$interrogatives,v4$rhetoricalquestion,v4$religion,v4$communities,v4$emotionaltone,v4$positiveemotions,v4$negativeemotions,v4$malereferences,v4$femalereferences,v4$festival,v4$leisure,v4$body,v4$health,v4$friends,v4$nonelite,v4$home,v4$simplewords,v4$shortsentences,v4$numbers,v4$cognitiveprocesses,v4$motion,v4$pastfocus,v4$certainty,v4$time,v4$festivalandculturalrefs,v4$conceptualnotions,v4$feat11)
colnames(allvars)<-c("institutionalprocesses","politicalparties","firstpersonsingular","firstpersonplural","thirdpersonsingular","thirdpersonplural","risk","assent","electoralprocesses","personalisedgovernance","family","interrogatives","rhetoricalquestion","religion","communities","emotionaltone","positiveemotions","negativeemotions","malereferences","femalereferences","festival","leisure","body","health","friends","nonelite","home","simplewords","shortsentences","numbers","cognitiveprocesses","motion","pastfocus","certainty","time","festivalandculturalrefs","conceptualnotions","feat11")
PMs<-as.factor(v4$PM)
resultnewanova <- aov(allvars~PMs)
summary(resultnewanova)
coefficients(resultnewanova)
newfvaluelists<-c()
for (f in 1:38){
newfvaluelists <- c(newfvaluelists,summary.aov(resultnewanova)[[f]][["F value"]][[1]])
}
newfvaluelists<-round(newfvaluelists,digits=2)
newfvaluelists
#2 Regrouping the variables of interest in 3 groups (well 4 atm)
popgroupvars <- cbind(populism,nonpopulism,neutral,shortwords)
colnames(popgroupvars)<-c("populism","nonpopulism","neutral","shortwords")
resultnewanova2 <- aov(popgroupvars~PMs)
summary(resultnewanova2)
coefficients(resultnewanova2)
newfvaluelists2<-c()
for (f in 1:4){
newfvaluelists2 <- c(newfvaluelists2,summary.aov(resultnewanova2)[[f]][["F value"]][[1]])
}
newfvaluelists2<-round(newfvaluelists2,digits=2)
newfvaluelists2
#3 Presenting the results PM-wise
v6<-aggregate(v4, by=list(v4$PM),FUN=mean,na.action = na.omit)
v6$PM <- NULL
v6$Year <- NULL
v6$Speech <- NULL
colnames(v6)[1]<-"PM"
v7<-apply(v6[,-(1)], 1, sum)
v8 <- cbind(PM,popgroupvars)
v9<-as.data.frame(v8)
colnames(v9)[1]<-"PM"
v9$PM <- factor(v9$PM,
levels = c(7,4,3,2,8,11,1,9,10,5,6),
labels = c("nehru","indira","desai","charan","rajiv","vpsingh","chandra","rao","vajpayee","mms","modi"))
v10<-aggregate(v9, by=list(v4$PM),FUN=mean,na.action = na.omit)
v10$PM <- NULL
colnames(v10)[1]<-"PM"
v11<-apply(v10[,-(1)], 1, sum)
scatter.smooth(x=v10$PM, y=v10$populism, main="PM ~ % populist tokens")
#Populismp<-as.matrix(v10$populism)
#colnames(Populismp)<-v10$PM
barplot(v10$populism-0.25, names.arg=c(v10$PM),main="populism", sub="(stylistic features)", #we took 0.25 from every single value in order to have the histogram start where we wanted #####FIGURE 1
xlab="Prime Ministers", ylab="% of tokens", axes=FALSE)
axis(2, at=c(0,0.02,0.04,0.06), labels=c(0.25,0.27,0.29,0.31)) #we add 0.25 back on
plot(v10$PM, v10$nonpopulism, main="Populist averse", sub="(stylistic features)",
xlab="Prime Ministers", ylab="% of tokens")
plot(v10$PM, v10$neutral, main="Neutral", sub="(stylistic features)",
xlab="Prime Ministers", ylab="% of tokens")
plot(v10$PM, v10$shortwords, main="Shortwords", sub="(stylistic features)",
xlab="Prime Ministers", ylab="% of tokens")
#stacked barplot
v10t <- as.matrix(t(v10))
colnames(v10t)<-v10t[1,]
v10t<-v10t[-1,]
barplot(v10t, legend=rownames(v10t))
#ggplot(v10, aes(x = `PM`)) +
#geom_bar() +
#theme(axis.text.x = element_text(angle = 45, hjust = 1))
#qplot(v10$PM, v10$populism, geom="point")
barplot(v10$populism, names.arg=v10$PM, ylim=c(0.25,0.31), ylab="populism", xlab="PM")
#ggplot(v10, aes(x = PM, y = populism, fill = ind)) +
#geom_bar(position = "fill",stat = "identity") +
# or:
# geom_bar(position = position_fill(), stat = "identity")
#scale_y_continuous(labels = scales::percent_format())
#p4 <- ggplot() + geom_bar(aes(y = percentage, x = PM, fill = popgroupvars), data = v10,
#stat="identity")
#p4
#v6<-v4
#v6$PM<-as.character(as.numeric(v4$PM))
#v6$Speech<-as.character(as.numeric(v4$Speech))
#v6dfm<-as.dfm(v6)
#dfm_group(v6dfm, groups = "PM")
#Part 7: Ratio popuslist, averse populist vocabulary
#popgroupvars <- cbind(populism,nonpopulism,neutral,shortwords)
#colnames(popgroupvars)<-c("populism","nonpopulism","neutral","shortwords")
#First, the ratio is generated
v11 <- cbind(Year,popgroupvars)
v12<-as.data.frame(v11)
colnames(v12)[1]<-"Year"
ratio <- as.matrix(populism/nonpopulism)
ratio.all <- data.frame(v12,ratio)
#Second, the glm is computed on each dictionary entry separately
Time <- time(v4$Year)
lm.ts2 <- glm( Time ~ v4$institutionalprocesses+v4$politicalparties+v4$firstpersonsingular+v4$firstpersonplural+v4$thirdpersonsingular+v4$thirdpersonplural+v4$risk+v4$assent+v4$electoralprocesses+v4$personalisedgovernance+v4$family+v4$interrogatives+v4$rhetoricalquestion+v4$religion+v4$communities+v4$emotionaltone+v4$positiveemotions+v4$negativeemotions+v4$malereferences+v4$leisure+v4$body+v4$health+v4$friends+v4$nonelite+v4$home+v4$simplewords+v4$shortsentences+v4$numbers+v4$cognitiveprocesses+v4$pastfocus+v4$certainty+v4$time+v4$festivalandculturalrefs+v4$conceptualnotions, data=v4) #collinear variables dropped
summary(lm.ts2)
#Third, the glm is computed on the ratio
v13<-cbind(v4,ratio)
Time2 <- time(v13$Year)
lm.ts3 <- glm(Time2 ~ ratio, data=v13)
summary(lm.ts3)
plot(ratio~as.numeric(v13$Year),data=v13, ylim=c(1,7))
#Fourth, we separate modi from the others
orderpm<-v13[order(v13$PM),]
min(which(orderpm$PM=="modi"))
max(which(orderpm$PM=="modi"))
notmodi<-orderpm[-(1889:2740),]
ismodi<-orderpm[(1889:2740),]
Time3 <- time(as.numeric(ismodi$Year))
lm.ts4 <- lm(Time3 ~ ratio, data=ismodi)
summary(lm.ts4)
plot(ratio~as.numeric(ismodi$Year),data=ismodi)
plot(ratio~as.numeric(v13$Year),data=v13)
#Standard error of the mean
sem <- function(x){
sd(x)/sqrt(length(x))
}
#v16<-sem(v14[,43])
#Compute the mean of scores for each year
avplotyear<-c() #empty vector
sdplotyear<-c()
for(i in unique(v13$Year)){ #takes all the years mentioned once
allratiosperyear<-v13[v13[,3]==i,42] #find out all the row in column 42 with year == i
sdperyear<-sd(allratiosperyear)
averageplot<-sum(allratiosperyear)/length(allratiosperyear) #computes the average for each year
avplotyear<-rbind(avplotyear,c(i,averageplot))#binds year and averages
sdplotyear<-rbind(sdplotyear,c(i,sdperyear))#binds year and standard deviations
}
plot(ratio~as.numeric(v13$Year),data=v13, ylim=c(1,5),col="lightgrey") ####FIGURE 3B
points(avplotyear, pch=2, col="red")
lines(avplotyear, col="red")
lines(sdplotyear[,1],avplotyear[,2]+sdplotyear[,2],lty=2, col="red")
lines(sdplotyear[,1],avplotyear[,2]-sdplotyear[,2],lty=2, col="red")
#v14<-(v13, by=list(v13$Year),FUN=mean,na.action = na.omit)
#plot(ratiaggregateo~as.numeric(v14$Year),data=v14)
#lm.ts5 <- lm(as.numeric(v14$Year) ~ ratio, data=v14)
#summary(lm.ts5)
#popgroupvars <- cbind(populism,nonpopulism,neutral,shortwords)
#colnames(popgroupvars)<-c("populism","nonpopulism","neutral","shortwords")
#v15 <- cbind(v4$Year,popgroupvars)
#colnames(v15)[1]<-"Year"
#v15df<-as.data.frame(v15)
#v15$Year <- as.numeric(as.character(v15$Year))
#plot(populism~as.numeric(v15df$Year),data=v15df)
#v16<-aggregate(v15df, by=list(v15df$Year),FUN=mean,na.action = na.omit)
#plot(nonpopulism~as.numeric(v16$Year),data=v16)
#for(i in 1:nrow(v13)){
#for(j in 4:ncol(v13)){
#v4[i,j]<-v3[i,j]/sum(v3[i,(4:ncol(v3))]) #words per speech matlab... sum(v3[i,(4:ncol(v3) | each cell...v3[i,j]
#}
#}
#r2 <- r
#r2<-r2[-3601,]
#r2<-v2[-3579,]
#r2<-r2[-1582,]
#r2<-r2[-150,]
#v2<-r2[-117,]
4 Beyond the scope of the article
STM on the dictionary lists
#with the headers of the weighted dictionary
dicotokssubworkcorpus <- tokens_lookup(tokssubworkcorpus, popdicoH, levels=3, valuetype = 'glob', exclusive = TRUE, capkeys = FALSE, case_insensitive = FALSE)
dfmdicotokssubworkcorpus <- dfm(dicotokssubworkcorpus, remove_punct = FALSE, tolower = FALSE, dictionary_regex=TRUE, language = "english", stem = FALSE, clean = FALSE, verbose= TRUE)
dfm2stm <- convert(dfmdicotokssubworkcorpus, to="stm")
iostm<- stm(documents = dfm2stm$documents, vocab = dfm2stm$vocab,
K = 3, #the number of topics to be generated
max.em.its = 75, data = dfm2stm$meta, #number of iterations
init.type = "Spectral")
out<-prepDocuments(dfm2stm$documents,dfm2stm$vocab, dfm2stm$meta, lower.thresh=1, upper.thresh = 500)
#with the actual words of dicitonary
#popdicoHflat <-flatten_dictionary(popdicoH)
#listflatdicotokssubworkcorpus<-unlist(flatdicotokssubworkcorpus)
#head(listflatdicotokssubworkcorpus)
#flatdicotokssubworkcorpus <- tokens_lookup(tokssubworkcorpus, popdicoH, valuetype = 'glob', exclusive = TRUE, capkeys = FALSE, case_insensitive = FALSE)
#flatdicotokssubworkcorpus
g <- dfm_select(dfmtokssubworkcorpus, pattern=popdicoH, selection = c("keep"))
g2stm <- convert(g, to="stm")
giostm<- stm(documents = g2stm$documents, vocab = g2stm$vocab,
K = 10, #the number of topics to be generated
max.em.its = 75, data = g2stm$meta, #number of iterations
init.type = "Spectral")
out<-prepDocuments(g2stm$documents,g2stm$vocab, g2stm$meta, lower.thresh=1, upper.thresh = 500)
Cross-validation
#Usually cross-validation is used to assesses how well your model (eg linear regression with quadratic term ) explain the relation between the independent variable (PM) and populism (dictionary). In this case however, we want to know how well the dictionary explains the variation in speech amongs speakers.
#1: cross-validation on the lm (year as ind. var and unified populism score as dep. var)
n <- 4156
folds <- sample(1:2, n, replace=TRUE)
pop <- as.numeric(as.character(z$Populism))
sal <- as.numeric(z$Year)
dat <- data.frame(sal,pop,folds)
fitting1 <- lm(pop~ sal, data=subset(dat, folds==1))
predict1 <- predict(fit, newdata=subset(dat, folds==2))
p.hat.fold2 <- as.numeric(predict1)
fitting2 <- lm(pop~ sal, data=subset(dat, folds==2))
predict2 <- predict(fit, newdata=subset(dat, folds==1))
p.hat.fold1 <- as.numeric(predict2)
dat$p.hat[dat$fold==2] <- p.hat.fold2
dat$p.hat[dat$fold==1] <- p.hat.fold1
rmse1 <- sqrt(mean((dat$p.hat-dat$p)^2))
rmse1
#2: cross-validation on the lm (pm as ind. var and various populist scores as dep. var)
n <- 4156
folds <- sample(1:2, n, replace=TRUE)
#StanValue5r<-as.data.frame(StanValue5)
#StanValue5r$document <- NULL
#StanValue5r <- as.numeric(as.character(StanValue5r))
e <- cbind(r$institutionalprocesses,r$politicalparties,r$firstpersonsingular,r$firstpersonplural,r$thirdpersonsingular,r$thirdpersonplural,r$risk,r$assent,r$electoralprocesses,r$personalisedgovernance,r$family,r$interrogatives,r$rhetoricalquestion,r$religion,r$communities,r$emotionaltone,r$positiveemotions,r$negativeemotions,r$malereferences,r$leisure,r$body,r$health,r$friends,r$nonelite,r$home,r$simplewords,r$shortsentences,r$numbers,r$cognitiveprocesses,r$pastfocus,r$certainty,r$time,r$festivalandculturalrefs,r$conceptualnotions)
colnames(r)[1]<-"Year"
colnames(r)[2]<-"PM"
m <- as.numeric(as.factor(r$PM))
n <- as.numeric(as.factor(r$Year))
e <- as.matrix(as.numeric(as.character(e)))
dat2 <- data.frame(m,e,folds)
fit2 <- lm(e ~ m, data=subset(dat2, folds==1))
pred2 <- predict(fit2, newdata=subset(dat2, folds==2))
e.hat.fold2 <- as.numeric(pred2)
fit3 <- lm(e ~ m, data=subset(dat2, folds==2))
pred3 <- predict(fit2, newdata=subset(dat2, folds==1))
e.hat.fold1 <- as.numeric(pred3)
dat2$e.hat[dat2$fold==2] <- e.hat.fold2
dat2$e.hat[dat2$fold==1] <- e.hat.fold1
rmse2 <- sqrt(mean((dat2$e.hat-dat2$e)^2))
rmse2
#cross-validation on the lm (speaker as ind. var and various weighted populist frequencies as dep. var)
n <- 4156
folds <- sample(1:2, n, replace=TRUE)
w <- as.matrix(as.numeric(as.character(popdicodfmHspeechWeight)))
l <- as.numeric(as.factor(popdicodfmHspeechWeight@docvars$loc))
dat3 <- data.frame(l,w,folds)
fit3 <- lm(w ~ l, data=subset(dat3, folds==1))
Cluster analysis
In a DFM, similarites of documents (clustering) are calculated. For more on the command, c.f.https://tutorials.quanteda.io/statistical-analysis/dist/
tstatdist <- as.dist(textstat_dist(dfmperpm))
clust <- hclust(tstatdist)
plot(clust, xlab = "Distance", ylab = NULL)
Lexical diversity
In a DFM, lexical diversity is calculated. That requires removing stopwords. For more on the command, https://tutorials.quanteda.io/statistical-analysis/lexdiv/.
dfmnostoptokssubworkcorpus <- dfm(ngramstokssubworkcorpus, remove = stopwords('en'), remove_punct = TRUE)
dfmperpmnostop <- dfm_group(dfmnostoptokssubworkcorpus, groups = "loc")
tstatlexdiv <- textstat_lexdiv(dfmperpmnostop)
tail(tstatlexdiv, 5)
plot(tstatlexdiv$TTR, type = 'l', xaxt = 'n', xlab = NULL, ylab = "TTR")
grid()
axis(1, at = seq_len(nrow(tstatlexdiv)), labels = docvars(dfmperpmnostop, 'loc'))
Top frequencies
In a DFM, highest frenquencies are plotted after removing puntuation and stopwords. For more on the command, https://tutorials.quanteda.io/statistical-analysis/frequency/.
freqdfmtokssubworkcorpusnostop <- textstat_frequency(dfmnostoptokssubworkcorpus, n = 20, groups = "loc")
head(freqdfmtokssubworkcorpusnostop, 100)
dfmperpmnostop %>%
textstat_frequency(n = 15) %>%
ggplot(aes(x = reorder(feature, frequency), y = frequency)) +
geom_point() +
coord_flip() +
labs(x = NULL, y = "Frequency") +
theme_minimal()
dfmloctokssubworkcorpus <- dfm(ngramstokssubworkcorpus, groups = "loc", remove = stopwords('en'), remove_punct = TRUE, clean = TRUE, stem = TRUE)
set.seed(132)
FCM
In a DFM, an analysis of similitudes is generated. In quanteda, it is called a co-occurrence matrix (FCM). For more on the command,https://tutorials.quanteda.io/basic-operations/fcm/fcm/.
dfmperpmtrim <- dfm_trim(dfmnostoptokssubworkcorpus, min_termfreq = 100)
topfeatures(dfmperpmtrim)
fcmatdfmtokssubworkcorpustrim <- fcm(dfmperpmtrim)
dim(fcmatdfmtokssubworkcorpustrim)
feat <- names(topfeatures(fcmatdfmtokssubworkcorpustrim, 50))
selectfcmatdfmtokssubworkcorpustrim <- fcm_select(fcmatdfmtokssubworkcorpustrim, pattern = feat)
dim(selectfcmatdfmtokssubworkcorpustrim)
size <- log(colSums(dfm_select(dfmperpmtrim, feat)))
set.seed(144)
textplot_network(selectfcmatdfmtokssubworkcorpustrim, min_freq = 0.8, vertex_size = size / max(size) * 3)
Cooccurrents
In DFM, we look at target-word collocations, that is cooccurrence in TXM. If used with the pattern “phrase”, we can bind together several pivot words. For more on the command, https://tutorials.quanteda.io/advanced-operations/target-word-collocations/.
nostoptokssubworkcorpus <- tokens_select(ngramstokssubworkcorpus, pattern = stopwords('en'), selection = 'remove')
notpuncnostoptokssubworkcorpus <- nostoptokssubworkcorpus %>% tokens_remove('[\\p{P}\\p{S}]', valuetype = 'regex', padding = TRUE)
india <- c('India*', 'Bharat', 'Hindustan')
toksIndia <- tokens_keep(notpuncnostoptokssubworkcorpus, phrase(india), window = 10)
toksnoIndia <- tokens_remove(notpuncnostoptokssubworkcorpus, phrase(india), window = 10)
dfmatIndia <- dfm(toksIndia)
dfmatIndiaperpm <- dfm_group(dfmatIndia, groups = "loc")
dfmatnoIndia <- dfm(toksnoIndia)
dfmatnoIndiaperpm <- dfm_group(dfmatnoIndia, groups = "loc")
tstatkeyIndia <- textstat_keyness(rbind(dfmatIndiaperpm, dfmatnoIndiaperpm), seq_len(ndoc(dfmatIndiaperpm)))
tstatkeyIndiasubset <- tstatkeyIndia[tstatkeyIndia$n_target > 10, ]
head(tstatkeyIndiasubset, 50)
Targeted dictionary analysis: other ways to plot populism
This would work better if the variable was time. For more on the command, https://tutorials.quanteda.io/advanced-operations/targeted-dictionary-analysis/.
dfmattoksIndiapopDfm <- dfm(toksIndia, dictionary = popdicoH) %>%
dfm_group(group = 'loc', fill = TRUE)
matplot(dfmattoksIndiapopDfm, type = 'l', xaxt = 'n', lty = 1, ylab = 'Frequency')
for(i in 1:11)
{ segments(i,0,i,FreqValues[i]) }
pm<-as.character(docvars(popdicodfmHpm, "loc"))
pm <- recode(pm, nehru = "J.Nehru", indira= "I.Gandhi", desai="M.Desai",charan="C.Singh", rajiv="R.Gandhi", vpsingh="VP.Singh", chandra="C.Shekhar", rao="PVN.Rao", vajpayee="AB.Vajpayee", mms="M.Singh", modi = "N.Modi")
axis(1,at=c(1:11),labels=pm, las=0)
n_india <- ntoken(dfm(toksIndia, group = docvars(toksIndia, 'loc')))
plot((dfmattoksIndiapopDfm[,2] - dfmattoksIndiapopDfm[,1]) / n_india,
type = 'l', ylab = 'Populism', xlab = '', xaxt = 'n')
for(i in 1:11)
{ segments(i,0,i,FreqValues[i]) }
pm<-as.character(docvars(popdicodfmHpm, "loc"))
pm <- recode(pm, nehru = "J.Nehru", indira= "I.Gandhi", desai="M.Desai",charan="C.Singh", rajiv="R.Gandhi", vpsingh="VP.Singh", chandra="C.Shekhar", rao="PVN.Rao", vajpayee="AB.Vajpayee", mms="M.Singh", modi = "N.Modi")
axis(1,at=c(1:11),labels=pm, las=0)
Classification: Rainert Method
The package is used to classify documents. Nb: The package is quite unstable. For more on the command, https://juba.github.io/rainette/articles/introduction_usage.html.
library(rainette)
dtm <- dfm_trim(dfmnostoptokssubworkcorpus, min_termfreq = 3)
res <- rainette(dtm, k = 5, min_uc_size = 10, min_members = 10)
rainette_explor(res, dtm)
Topic Modelling
Here topics are computed using the package stm. For more on the commands, https://www.rdocumentation.org/packages/stm/versions/1.3.3/topics/stm
dfm2stm <- convert(dfmperpmnostop, to="stm")
iostm<- stm(documents = dfm2stm$documents, vocab = dfm2stm$vocab,
K = 10, #the number of topics to be generated
max.em.its = 75, data = dfm2stm$meta, #number of iterations
init.type = "Spectral")
out<-prepDocuments(dfm2stm$documents,dfm2stm$vocab, dfm2stm$meta, lower.thresh=1, upper.thresh = 500) #upper.tresh will remove tokens if they appear in more than X number of documents
iostm<- stm(documents = out$documents, vocab = out$vocab,
K = 10,
max.em.its = 75, data = out$meta,
init.type = "Spectral")
#iostm2<- stm(documents = out$documents, vocab = out$vocab,
#K = 10, prevalence =~ volume, #'volume' is a ponderation variable
#max.em.its = 75, data = out$meta,
#init.type = "Spectral")
ioSelect <- selectModel(out$documents, out$vocab, K = 10,
max.em.its = 75,
data = out$meta, runs = 20, seed = 020309) # check seeds once
stm.many<-searchK(out$documents, out$vocab, K = c(8:12),
data = out$meta)
labelTopics(iostm)
plot(iostm, type = "summary", xlim = c(0, .3))
#That can work only if the original dataframe and the dfm from which the analysis is performed are or the same lenght
thoughts3 <- findThoughts(iostm, texts = dataframe2$text, #Outputs most representative docs for a particular topic.
n = 2, topics = 10)$docs[[1]]
mod.out.corr<-topicCorr(iostm)
plot(mod.out.corr)
Wordsfish
Here we can scale documents without having to use reference scores. For more on the command, c.f. https://tutorials.quanteda.io/machine-learning/wordfish/.
tmod_wf <- textmodel_wordfish(dfmperpmnostop, dir = c(6,5))
summary(tmod_wf)
textplot_scale1d(tmod_wf)
textplot_scale1d(tmod_wf, groups = docvars(dfmat_irish, "typegeneral"))
textplot_scale1d(tmod_wf, margin = "features",
highlighted = c("brothers", "sisters", "children",
"bank", "economy", "the", "citizenship",
"productivity", "deficit"))
Specificities TXM
Display specificities probability distribution. For more on the command, https://rdrr.io/cran/textometry/man/specificities.distribution.plot.html
plotspecif <- specificities.distribution.plot(7799, 22957, 2199993, 8029517)
#plotspecif <- specificities.distribution.plot(x, F, t, T)
#x: observed number of A words
#F: total number of A
#t: size of part
#T: size of corpus
Correspondence Analysis
#Case 1: we look at PMs year-wise using a predefined variable in the medadata.csv
dfmnostoptokssubworkcorpus <- dfm(ngramstokssubworkcorpus, remove = stopwords('en'), remove_punct = TRUE)
dfmperpmyearnostop <- dfm_group(dfmnostoptokssubworkcorpus, groups = "pmyear")
tmod_ca <- textmodel_ca(dfmperpmyearnostop)
textplot_scale1d(tmod_ca)
dat_ca <- data.frame(dim1 = coef(tmod_ca, doc_dim = 1)$coef_document,
dim2 = coef(tmod_ca, doc_dim = 2)$coef_document)
head(dat_ca)
plot(1, xlim = c(-1.5, 6), ylim = c(-2, 2), type = 'n', xlab = 'Dimension 1', ylab = 'Dimension 2')
grid()
text(dat_ca$dim1, dat_ca$dim2, labels = rownames(dat_ca), cex = 0.8, col = rgb(0, 0, 0, 0.7))
#Case 2: We look at vocabulary-wise data through transpose...but better results with the combination of clustering and CA in Iramuteq
subworkcorpusmodi<-corpus_subset(workcorpus, format %in% c('speech') & loc %in% c('modi'))
tokssubworkcorpusmodi <- tokens(subworkcorpusmodi, remove_punct = FALSE, remove_numbers = FALSE, remove_symbols = FALSE, remove_separators = TRUE, remove_hyphens = FALSE, remove_url = FALSE, concatenator = "_")
head(tokssubworkcorpusmodi[[1]], 50)
ngramstokssubworkcorpusmodi <- tokens_lookup(tokssubworkcorpusmodi, dicopopgram, valuetype = 'glob', exclusive = FALSE, capkeys = FALSE, case_insensitive = FALSE)
dfmtokssubworkcorpusmodi <- dfm(ngramstokssubworkcorpusmodi, remove_punct = TRUE, tolower = TRUE, dictionary_regex=TRUE, language = "english", stem = TRUE, clean = TRUE, verbose= TRUE)
dfmmoditrim <- dfm_trim(dfmtokssubworkcorpusmodi, min_termfreq = 100)
dfmmoditrimasdf <- convert(dfmmoditrim, to = "data.frame")
dfmmodiasdftrans <- t(dfmmoditrimasdf)
colnames(dfmmodiasdftrans) <- as.character(unlist(dfmmodiasdftrans[1,]))
dfmmodiasdftrans <- dfmmodiasdftrans[-1, ]
#all fine till here...but then the dataframe is not recognized correctly
#once fixed:
#x<-CA(dfmmodiasdftrans)
#or follow step 1
#modivocab <-as.dfm(dfmmodiasdftrans)
#tmod_ca2 <- textmodel_ca(modivocab)
#textplot_scale1d(tmod_ca2)
Transpose
How to do this? https://stackoverflow.com/questions/3835280/pivoting-rows-into-columns
dfmperpmasdf <- convert(dfmperpm, to = "data.frame")
dfmperpmasdftrans <- t(dfmperpmasdf)
colnames(dfmperpmasdftrans) <- as.character(unlist(dfmperpmasdftrans[1,]))
dfmperpmasdftrans <- dfmperpmasdftrans[-1, ]
Lemmatization
It is possible to use an already existing dictionary to lemmatise the corpus. For more on the command, https://github.com/quanteda/quanteda/issues/1022, lemma dictionary us available here: https://github.com/michmech/lemmatization-lists
data <- read.csv("lemmatization/lemmatization-en.txt", sep = '\t', as.is = TRUE,
header = FALSE)
dict <- dictionary(split(data[,2], data[,1]))
tokslemmasubworkcorpus <- tokens_lookup(ngramstokssubworkcorpus, dict, valuetype = 'fixed', exclusive = FALSE, capkeys = FALSE)
Word embedding
feats <- dfm(nostoptokssubworkcorpus, verbose = TRUE) %>%
dfm_trim(min_termfreq = 5) %>%
featnames()
padding <- tokens_select(nostoptokssubworkcorpus,feats, padding = TRUE)
tvecfcmnostoptokssubworkcorpus <- fcm(padding, context = "window", count = "weighted", weights = 1 / (1:5), tri = TRUE)
glove <- GlobalVectors$new(word_vectors_size = 50, vocabulary = featnames(tvecfcmnostoptokssubworkcorpus), x_max = 10)
corpus_main <- fit_transform(tvecfcmnostoptokssubworkcorpus, glove, n_iter = 20)
corpus_context <- glove$components
corpus_vectors <- as.dfm(corpus_main + t(corpus_context))
pesa <- corpus_vectors["money", ] -
corpus_vectors["bank", ] +
corpus_vectors["mantra", ]
cos_sim <- textstat_simil(corpus_vectors, pesa,
margin = "documents", method = "cosine")
head(sort(cos_sim[, 1], decreasing = TRUE), 20)
Here DFMs are exported to a csv for further analysis.
write.csv(dfmperpmasdftrans,file="C:/Users/jtmartelli/Google Drive/Textual_analysis/R/dfmperpmasdftrans.csv")
write.csv(dfmtokssubworkcorpus,file="C:/Users/jtmartelli/Google Drive/Textual_analysis/R/dfmtokssubworkcorpus.csv")